Press Release RBI Working Paper Series No. 09 State-Level Inflation Forecasts for India: Based on Data from Inflation Expectations Survey of Households Purnima Shaw and R. K. Sinha@ Abstract 1The Inflation Expectations Survey of Households (IESH) of the Reserve Bank of India (RBI) completed its 90th round in March 2024. Using the historical series from this survey, we provide state-level inflation forecasts, which can help in better understanding of inflation dynamics. Deviating from the conventional regression-based forecasting, this paper proposes a new approach to modelling inflation expectations, which not only uses centre-wise survey data and state-wise inflation data but also redistributes the inflation expectation of respondents suitably to gain further precision. For most of the states, the analysis suggests a noticeable reduction in the quantum of nowcast/ forecast errors in state-level nowcasts/ forecasts obtained using the proposed methodology when compared with the errors of the survey forecasts, bias-adjusted survey forecasts and linear regression-based forecasts. JEL Classification: D84, E31 Keywords: Inflation, inflation expectations, percentile, probability distribution function Introduction The information on inflation expectations is widely used by central banks for monetary policy making. Several central banks conduct high-frequency surveys to collect information on short to medium-term inflation perceptions. The Reserve Bank of India (RBI) conducts Survey of Professional Forecasters (SPF), Industrial Outlook Survey (IOS), Services and Infrastructure Outlook Survey (SIOS), Consumer Confidence Survey (CCS) and Inflation Expectations Survey of Households (IESH). In this paper, we focus on inflation expectations of households collected through IESH. IESH is conducted across approximately 6,000 respondents in 19 urban centres/ cities. The survey captures both qualitative and quantitative inflation perceptions of households. However, we restrict our analysis only to quantitative information in the paper, which is sought in the survey over three time-horizons: (i) current inflation perception, (ii) three-month ahead inflation expectations and (iii) one-year ahead inflation expectations. The frequency of IESH is six times per annum, aligning with the frequency2 of the bi-monthly Monetary Policy Committee meetings of the RBI. Econometric methods are commonly used for nowcasting and forecasting actual inflation based on inflation expectations data. Recently, Sinha (2023) demonstrated the potential use of statistical distributions to explain inflation and inflation expectations and linking them using suitable functions. The paper applied the method to all-India inflation and inflation expectations of all the survey centres together. Given India’s geographical diversity, each state differs in consumption patterns, which can result in variations in inflation and inflation expectations. Other factors like inter-state transportation costs, differences in state tax regimes, varying freight costs, geographical distances, and state-level food supply management can also contribute to inflation variability. Hence, forecasting all-India level inflation may overlook the important state-specific information. In this paper, we extend Sinha’s (2023) work by applying it to centre-wise data of inflation and inflation expectations and provide state-level inflation nowcasts and forecasts. Additionally, to better analyse the round number preferences of the respondents in IESH, we propose a novel approach of decomposing inflation expectations into discrete and continuous data. The paper is divided into five sections. The second section reviews the relevant literature. The third section provides the methodology to split the datasets into discrete and continuous components distinctly and map these data with the inflation data to obtain state-level inflation nowcasts and forecasts. The numerical demonstration, illustrated in the fourth section, based on the proposed methodology evaluates the performance of the estimates in comparison with the actual inflation numbers. The last section concludes and provides a direction for future studies on the subject. II. Literature Review Inflation expectations generally tend to be higher than the realised inflation, a trend observed globally, including in advanced economies. Abildgren and Kuchler (2021) refer to this overestimation as the “inflation perception conundrum”. The upward bias in households’ reporting of inflation perceptions and expectations may not be a major concern if it is consistent, and econometric models can filter this out when forecasting actual inflation. However, the concern becomes more serious when the bias is inconsistent and fluctuates unpredictably over time, without being explained by business cycles or economic outlooks. Recently, Singh et al. (2024) conducted a study on the nature of bias in inflation expectations in India and in other economies and found that the characteristics of the data in India were similar to those in other economies. They also explored another potential use of the survey - its relation with households’ future savings - and established a link. Inflation expectations can vary across households based on their socio-economic and demographic characteristics [Jonung (1981), Souleles (2004), Malmendier and Nagel (2016), Goyal and Parab (2019), Goldfayn-Frank and Wohlfart (2020) and Shaw (2024)]. The observed probability distributions of inflation perceptions and expectations tend to reveal peaks at specific points, usually in multiples of five, indicating the round number preference of respondents when expressing their opinions. Some recent studies, such as Krifka (2009), Binder (2017) and Reiche and Meyler (2022), have considered this behaviour an indicator of uncertainty regarding inflation. Krifka (2009) described this through a principle, namely, Round Numbers Round Interpretation Principle. According to this principle, short and simple numbers are related to low accuracy, whereas long and complex numbers correspond to higher accuracy. Binder (2017) developed an uncertainty index based on the frequency of round numbers, suggesting that a number is considered uncertain if it is divisible by five. Reiche and Meyler (2022) found that this uncertainty increases during the periods of economic instability. The probability distribution of a stochastic process is given by the probability that a realised value of the variable falls in a specified range. An empirical probability distribution of the stochastic series is obtained by finding the observed frequency distribution. Researchers have also explored the fitting of probability distributions to time series data. For instance, Osborne (1959) analysed the probability distribution of share price changes using the New York Stock Exchange data.3 Later, Praetz (1972) extended Osborne’s (1959) theory by incorporating the dynamic nature of volatility. More recently, Qiao et al. (2022) proposed a distribution coupling the distribution of extreme values of time series data with a distribution for frequently occurring values. Departing from conventional regression-based nowcasting and forecasting techniques, Sinha (2023) fitted statistical probability distributions to inflation and inflation expectations data for India, providing inflation forecasts by relating the two distributions. In the current paper, the approach of Sinha (2023) is utilised and generalised to map the datasets of inflation and inflation expectations after fitting them separately with suitable probability distributions in each of the centres. To manage centre-wise data, which tend to be uneven due to respondents’ preference for round numbers, a novel method is deployed. This method reduces additional frequency observed at peaks associated with round numbers by removing the interpolated frequencies based on the frequencies of the neighbouring points. In doing so, the probability density function is adjusted for better fitting, and the removed values are reassembled into a new discrete dataset representing a probability mass function. The detailed methodology is described in the following section. III. Methodology While upward bias (definition provided in Table A3) in the inflation expectations data when compared with the realised Consumer Price Index (CPI) – Urban inflation (CPI-U) figures is well-accepted, it may be of interest to look at the centre-wise biases (Tables 1 to 3). This analysis is performed with the assumption that the centre-wise inflation expectations represent the corresponding state’s urban inflation forecasts. These data indicate that the biases not only vary widely across the states, but they also fluctuate considerably across time. Thus, the biases in the centre-wise inflation expectations data appear to be dynamic. Hence, for deriving the inflation nowcasts and forecasts using the survey data, a centre-wise analysis seems more relevant.
Table 1: Inflation Perceptions as Nowcasts and Realised CPI-U Inflation |
State |
Maximum Bias |
Minimum Bias |
State |
Maximum Bias |
Minimum Bias |
Gujarat |
12.0 |
0.7 |
West Bengal |
10.0 |
-0.8 |
Karnataka |
5.3 |
-1.9 |
Uttar Pradesh |
8.8 |
-1.5 |
Madhya Pradesh |
6.8 |
-0.1 |
Maharashtra (using Mumbai) |
7.6 |
1.2 |
Odisha |
10.1 |
-1.6 |
Maharashtra (using Nagpur) |
10.7 |
-0.2 |
Tamil Nadu |
9.2 |
0.2 |
Bihar |
7.8 |
-3.2 |
Delhi |
8.9 |
-0.1 |
Kerala |
8.4 |
-0.7 |
Assam |
11.4 |
-2.8 |
Chandigarh |
8.5 |
-1.3 |
Telangana |
7.3 |
-0.7 |
Jharkhand |
6.3 |
-1.9 |
Rajasthan |
7.9 |
-1.6 |
Chhattisgarh |
8.4 |
-1.5 |
Note: The survey centre Jammu is added to the list of IESH centres from March 2021 round onwards. Hence, due to insufficient data, the centre is omitted from this analysis. Source: Authors’ calculations. |
Table 2: Three-month ahead Inflation Expectations as Forecasts and Realised CPI-U Inflation |
State |
Maximum Bias |
Minimum Bias |
State |
Maximum Bias |
Minimum Bias |
Gujarat |
12.7 |
1.8 |
West Bengal |
9.6 |
-0.7 |
Karnataka |
7.5 |
-1.5 |
Uttar Pradesh |
11.1 |
0.5 |
Madhya Pradesh |
8.5 |
0.9 |
Maharashtra (using Mumbai) |
8.8 |
1.4 |
Odisha |
11.9 |
-1.5 |
Maharashtra (using Nagpur) |
10.8 |
0.9 |
Tamil Nadu |
9.0 |
2.1 |
Bihar |
10.4 |
-2.6 |
Delhi |
9.4 |
-0.6 |
Kerala |
6.9 |
-0.4 |
Assam |
12.3 |
-4.0 |
Chandigarh |
9.3 |
-0.7 |
Telangana |
8.7 |
-1.3 |
Jharkhand |
6.7 |
-1.1 |
Rajasthan |
8.5 |
-0.7 |
Chhattisgarh |
10.8 |
-1.6 |
Note: The survey centre Jammu is added to the list of IESH centres from March 2021 round onwards. Hence, due to insufficient data, the centre is omitted from this analysis. Source: Authors’ calculations. |
Table 3: One-Year Ahead Inflation Expectations as Forecasts and Realised CPI-U Inflation |
State |
Maximum Bias |
Minimum Bias |
State |
Maximum Bias |
Minimum Bias |
Gujarat |
15.4 |
2.0 |
West Bengal |
11.2 |
-1.2 |
Karnataka |
8.3 |
-1.1 |
Uttar Pradesh |
12.3 |
0.6 |
Madhya Pradesh |
10.9 |
0.3 |
Maharashtra (using Mumbai) |
8.7 |
-0.6 |
Odisha |
12.7 |
-1.3 |
Maharashtra (using Nagpur) |
12.9 |
-1.3 |
Tamil Nadu |
13.3 |
2.4 |
Bihar |
13.7 |
-4.5 |
Delhi |
9.9 |
0.8 |
Kerala |
11.8 |
-0.1 |
Assam |
13.1 |
-5.3 |
Chandigarh |
14.0 |
0.2 |
Telangana |
9.6 |
0.1 |
Jharkhand |
8.7 |
-3.0 |
Rajasthan |
10.2 |
-2.5 |
Chhattisgarh |
8.3 |
-1.6 |
Note: The survey centre Jammu is added to the list of IESH centres from March 2021 round onwards. Hence, due to insufficient data, the centre is omitted from this analysis. Source: Authors’ calculations. |
We then study the state-wise official inflation data and centre-wise survey data by fitting appropriate probability distributions to them separately, and then linking the two through fitted distributions. Fitting probability distributions to the inflation and survey data facilitate a complete study of the data characteristics, including moments, skewness, kurtosis, etc. Let x be a real variable denoting the official inflation series of a state for a finite number of time periods T. While the aggregate inflation data may follow standard probability distribution or a mixture of two standard probability distributions (Sinha, 2023), the state-wise data are expected to display greater variability. As a result, to fit a probability distribution to the state-wise official inflation series, several continuous probability distributions are fitted to the data and it may be better to consider that x is fitted with a mixture of say, n probability distributions. The practice of using mixture probability distributions is popular in the literature. Johnson et al. (1994) described a theory on deriving a two-piece Normal distribution in which half of the pieces of two different Normal distributions with the same mode, but different standard deviations were joined together. Blix and Sellin (1998) used a two-piece Normal distribution to incorporate asymmetric risks to forecasts. Banerjee and Das (2011) also followed the same to apply in the Indian case. Sinha (2023) refers to literature like Cooray and Ananda (2005), Scollnik (2007), Ciumara (2006), Scollnik and Sun (2012), Nadarajah and Bakar (2014) and Frigessi et al. (2002) to fit a set of two probability distributions to the inflation data in India. The probability distribution function of x may, thus, be denoted as,
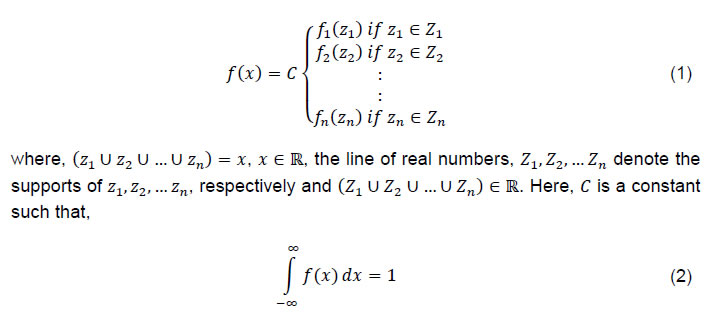
We estimate the probabilities for each of the inflation brackets using twelve probability distributions and choose the best fit for each bracket. In such a case, calculating the goodness of fit measure may not be of much relevance. The distribution function of x for any real number "a" is defined as,
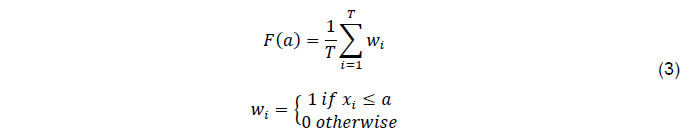
Here, F(a) denotes the proportion of x-values in the finite time frame not exceeding a real number "a". An important characteristic of the inflation sentiment data in India is the respondents’ preference for round numbers in polling their expectations (Sinha, 2023). This characteristic is expected to be even more prominent in the centre-wise data. This makes the centre-wise survey data uneven which makes the task of fitting suitable probability distribution extremely difficult. Hence, in fitting a suitable probability distribution to the state-wise inflation expectations survey dataset, an approach similar to that in fitting probability distribution to the official inflation dataset may not be appropriate. For the ease of handling the data, it is intended to classify the survey data into two portions in which the first portion would only display the dataset due to digit preference and the second portion would consist of the remaining dataset displaying a much smoother distribution. Although the survey data is continuous, the first portion, due to digit preference, is discrete, and the remaining portion remains continuous. To perform this data segregation, we consider the survey data for a finite time period. As per the survey questionnaire, the quantitative inflation sentiments of consumers are collected in the range of (<1%,1−<2%,…,15−<16%,≥16%). Respondents who answer “≥16 per cent” are advised to provide the exact inflation perception/ expectation number. Let y be a real variable, taking values yk representing the inflation sentiment of the consumers. The variable yk takes value labels in the set V = (< 1%, 1−< 2%,…,j,…15−< 16%, 16%, 17%, 18%,….) in the survey data. As observed from the IESH data, yk is allowed to take decimal values. Further, this is a variable indicating the sentiments on inflation, which itself is a continuous variable. Hence, it can be safely concluded that yk is a continuous variable. Let the frequency distribution of this variable be represented as (yk,fk), where fk is the frequency of yk observed from the data of a survey centre. Now, to segregate the frequency distribution of the original continuous data into discrete and continuous data at first, gk is computed as-
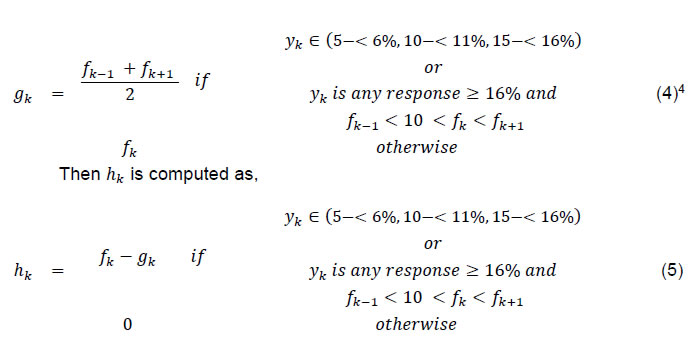
Here, the frequency distribution (yk,gk) denotes the new frequency distribution of continuous data on yk. The frequency values gk are calculated with the logic that at every yk, wherever there is a peak in frequency due to consumers’ preference for certain digits, the frequency for the continuous data is estimated by interpolation method. This segregation from continuous raw survey data to discrete and continuous survey data results in a much smoother distribution for the continuous data, thus making it much easier to fit appropriate probability distributions.
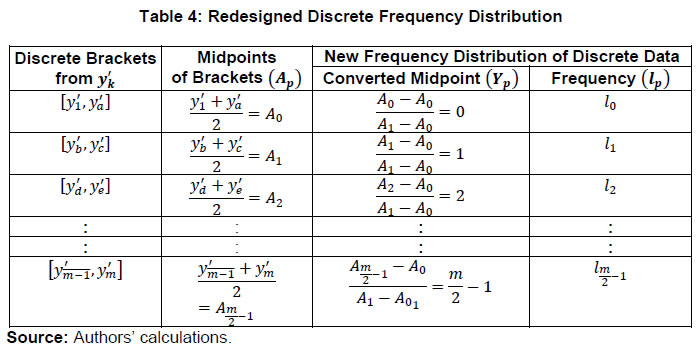
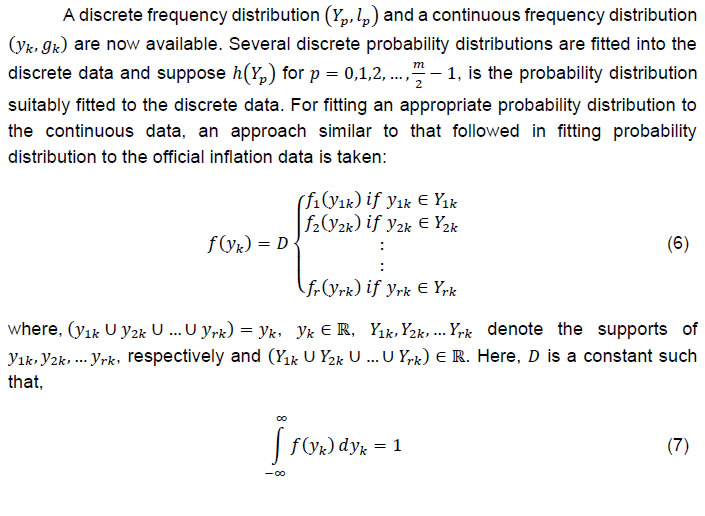
Now, once f(x), its related distribution function F(a) at a, h(yp) and f(yk) and the estimates of the related parameters of the fitted distributions are available, the idea now is to revert the estimated probabilities of the survey figures to the corresponding percentiles of the distribution function of the official inflation data. The statistical moments of the two distributions are very different and mapping the two distributions facilitates a correspondence between the two. Various Copula functions can be applied for this mapping. Sinha (2023) explains direct and indirect mapping procedures and executes the direct mapping method. In this paper, we explore finding the quantile of the inflation distribution for a given probability in the distribution for inflation expectations as follows:
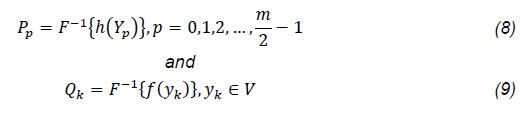
The survey-based expectations are biased towards larger numbers as compared to the official inflation figures. Hence, it may be assumed that the distribution of the inflation expectations is shifted towards the right of the distribution of the official inflation. In the survey distribution, if the probability of expecting a higher inflation number increases, then the corresponding percentile of the inflation data will be towards a number higher than the previously realised inflation figure. As distributions of the official inflation data and the survey data are widely different (apparent from the bias in the survey data), a mapping of the distributions is expected to remove the bias in the survey data and present refined inflation forecasts, namely, Pp from the discrete5 survey data, Qk from the continuous survey data and Pp + Qk as a forecast obtained from the combined survey data. We observe that the discrete survey data on inflation perceptions, three-month ahead and one-year ahead inflation expectations contain more information on the realised official inflation figures than the continuous survey data on the inflation sentiments. Taking this into account, the proposed inflation estimates are restricted to Pp. We also consider the estimates obtained from the sum Pp + Qk as alternative inflation estimates. Usually, time-varying coefficients are estimated in econometric models for nowcasting and forecasting inflation. This is important for updating the nowcasts and forecasts with the recent changes in the economy. Accordingly, with the addition of new datasets on inflation expectations (from survey rounds) and realised CPI-U inflation, we update the probability distributions of both datasets by following the same method of finding the best-fit distribution out of 12 distributions for each of the data brackets. Thus, crucial information on the time-varying nature of both datasets is retained in the derived nowcasts and forecasts. The distributions to be studied are kept fixed throughout the paper (one may add other distributions to the set and explore for better fit), thus eliminating the possibility of subjectivity in distribution fitting, if any. IV. Nowcasting/ Forecasting State-level Urban Inflation To examine the applicability of the above methodology, at first, the state-wise CPI-U6 general7 inflation data8, pertaining to states9 in which the IESH is conducted, is considered from January 2014 to December 2023. The state-wise IESH unit-level data10, are used from December 2013 to September 2023. The information on exact inflation perceptions and expectations (for responses labelled '≥16 per cent') are available in a consistent manner from the December 2013 onwards. Our objectives are to use the inflation perceptions’ survey data to nowcast the CPI-U inflation of the survey period and also to use the three-month ahead and one-year ahead inflation expectations’ survey data to forecast the CPI-U inflation of the months which are three-month ahead and one-year ahead of the survey month, respectively. To perform this exercise, available information on the CPI-U inflation of the months just prior to the survey month is used to compute the out-of-sample inflation forecasts. The out-of-sample nowcast estimates and two types of forecast estimates are obtained for 20 months each. To obtain out-of-sample nowcasts and forecasts of CPI-U inflation using the survey data, mapping of the survey months and nowcast and forecast months is performed as shown in Table 5.
Table 5: Mapping of Survey Periods CPI-U Nowcasting/ Forecasting Months |
Current |
Three-months ahead |
One-year ahead |
Survey Month |
CPI-U Nowcast Month |
Survey Month |
CPI-U Forecast Month |
Survey Month |
CPI-U Forecast Month |
July 2020 |
July 2020 |
July 2020 |
Oct. 2020 |
Nov. 2019 |
Nov. 2020 |
Sept. 2020 |
Sept. 2020 |
Sept. 2020 |
Dec. 2020 |
Jan. 2020 |
Jan. 2021 |
Nov. 2020 |
Nov. 2020 |
Nov. 2020 |
Feb. 2021 |
Mar. 2020 |
Mar. 2021 |
Jan. 2021 |
Jan. 2021 |
Jan. 2021 |
April 2021 |
May 2020 |
May 2021 |
Mar. 2021 |
Mar. 2021 |
Mar. 2021 |
June 2021 |
July 2020 |
July 2021 |
May 2021 |
May 2021 |
May 2021 |
Aug. 2021 |
Sept. 2020 |
Sept. 2021 |
July 2021 |
July 2021 |
July 2021 |
Oct. 2021 |
Nov. 2020 |
Nov. 2021 |
Sept. 2021 |
Sept. 2021 |
Sept. 2021 |
Dec. 2021 |
Jan. 2021 |
Jan. 2022 |
Nov. 2021 |
Nov. 2021 |
Nov. 2021 |
Feb. 2022 |
Mar. 2021 |
Mar. 2022 |
Jan. 2022 |
Jan. 2022 |
Jan. 2022 |
April 2022 |
May 2021 |
May 2022 |
Mar. 2022 |
Mar. 2022 |
Mar. 2022 |
June 2022 |
July 2021 |
July 2022 |
May 2022 |
May 2022 |
May 2022 |
Aug. 2022 |
Sep 2021 |
Sep 2022 |
July 2022 |
July 2022 |
July 2022 |
Oct. 2022 |
Nov. 2021 |
Nov. 2022 |
Sept. 2022 |
Sept. 2022 |
Sept. 2022 |
Dec. 2022 |
Jan. 2022 |
Jan. 2023 |
Nov. 2022 |
Nov. 2022 |
Nov. 2022 |
Feb. 2023 |
Mar. 2022 |
Mar. 2023 |
Jan. 2023 |
Jan. 2023 |
Jan. 2023 |
April 2023 |
May 2022 |
May 2023 |
Mar. 2023 |
Mar. 2023 |
Mar. 2023 |
June 2023 |
July 2022 |
July 2023 |
May 2023 |
May 2023 |
May 2023 |
Aug. 2023 |
Sept. 2022 |
Sept. 2023 |
July 2023 |
July 2023 |
July 2023 |
Oct. 2023 |
Nov. 2022 |
Nov. 2023 |
Sept. 2023 |
Sept. 2023 |
Sept. 2023 |
Dec. 2023 |
Jan. 2023 |
Jan. 2024 |
Sources: RBI, MOPSI and Authors’ calculations. |
We then examine the probability distribution of the CPI-U inflation series of each of the 18 states separately. On an experimental basis, the distribution-fitting exercise is initially conducted for a period of twelve months, i.e., from September 2022 to August 2023. Chart A1 displays these distributions of the CPI-U inflation data. These show that the distributions are somewhat smooth only for the states of Delhi, Assam, Bihar and Kerala. For the rest of the states, no single standard distribution can fit well into the observed probability distributions. The modal inflation lies in the range of 4 per cent to 7 per cent in most of the states. 17 per cent and 25 per cent of the inflation values in the case of Delhi and Chhattisgarh, respectively are less than or equal to 2 per cent; for the rest of the states under study for the mentioned period, there is no observation equal to or less than 2 per cent. However, more than half of the inflation values lie beyond 6 per cent in the case of Gujarat, Madhya Pradesh, Tamil Nadu, Telangana, Uttar Pradesh and Maharashtra. To fit distribution(s) to the CPI-U inflation data of each of the states under study, following Sinha (2023), 12 distributions, namely, Johnson SB, Cauchy, Burr, Laplace, Lognormal, Exponential, Gamma, Logistic, Weibull, Inverse Gamma, Loglogistic and Inverse Weibull are experimented. For each inflation bracket, the best-fit distribution (the distribution for which the fitted probability is nearest to the observed probability) is taken (Table A1). It is observed that for each of the 18 states under study, a mixture of probability distributions fit the data well. Now, following equations (1) and (2), for each state, the estimated probability distribution functions are proportioned into their respective weights and stitched together so that the final probability distribution function consisting of a mixture of probability distribution functions adds up to unity (Chart A1). Now, it is intended to fit distributions to the centre-wise inflation perceptions’ and expectations’ data of the IESH. First, a distribution is fitted to state-wise inflation expectations’ data of the September 2023 survey round. Due to the digit preferences of respondents in polling their inflation sentiments (observed as long spikes in observed frequencies for certain numbers in Chart A2) and using equations (4) and (5) and Table 4, the observed frequency distribution is broken into discrete and continuous frequency distributions. Table 6 provides the share of estimated discrete frequencies out of the total frequencies in each of the survey centres. The shares are comparatively higher in the perceptions about the current inflation than in the expectations about the future inflation over three months and one year. The shares are less than 50 per cent.
Table 6: Percentage of Discrete Responses in IESH September 2023 Survey Round |
Survey Centre |
Inflation Perceptions |
Three-month ahead Inflation Expectations |
One-year ahead Inflation Expectations |
Ahmedabad |
47.2 |
37.2 |
36.7 |
Bangalore |
34.9 |
26.0 |
26.3 |
Bhopal |
38.8 |
30.9 |
32.4 |
Bhubaneswar |
36.8 |
21.5 |
27.7 |
Chennai |
49.9 |
35.8 |
39.9 |
Delhi |
36.4 |
28.8 |
28.0 |
Guwahati |
42.0 |
37.5 |
34.4 |
Hyderabad |
32.1 |
24.3 |
30.0 |
Jaipur |
36.5 |
27.2 |
27.4 |
Jammu |
50.0 |
42.5 |
40.0 |
Kolkata |
42.7 |
36.5 |
34.0 |
Lucknow |
37.3 |
27.6 |
27.7 |
Mumbai |
31.8 |
25.7 |
25.6 |
Nagpur |
31.8 |
21.3 |
22.2 |
Patna |
23.6 |
14.0 |
18.7 |
Thiruvananthapuram |
47.4 |
32.4 |
24.7 |
Chandigarh |
28.6 |
18.5 |
18.4 |
Ranchi |
17.7 |
12.9 |
15.4 |
Raipur |
23.8 |
15.7 |
16.7 |
Sources: RBI and Authors’ calculations. |
In the case of the discrete frequency distribution segregated from the survey frequency distribution, Poisson distribution and Negative Binomial distributions are fitted. For the September 2023 survey round, the Poisson distribution fits the data well. The estimated values of the parameter λ for the fitted Poisson(λ) distribution are provided in Table A2. The continuous data is also fitted with appropriate probability distributions using equations (6) and (7). Frequency peaks are also observed at <1 per cent response option for the one-year ahead inflation expectations but not in the three-month ahead inflation expectations. This reflects consistency in the responses because for respondents who expect prices to remain the same/ decline (responses to qualitative questions) in the next year as compared to the current period (about 12 per cent in the study period), the quantitative inflation expectations must (by definition) be <1 per cent (about 8 per cent in the study period) as no price change (price decrease) over a year implies zero inflation (deflation). So, this phenomenon in the responses is a required criterion as per the structure of the questionnaire, rather than being noise in the data, as is the case with digit preference. With this logic, the frequency peaks at <1 per cent in the one-year ahead inflation expectations are retained. The state-wise observed frequency distributions and fitted probability distributions for both the discrete and continuous data portions of the one-year-ahead inflation expectations in the September 2023 survey round are displayed in Chart A3. From Charts A2 and A3, it is clear that frequent high spikes in the observed frequency distributions of the inflation expectations are now separated into discrete data, and the remaining data are comparatively smoother, thus making the task of fitting distributions easier. Now, following the above procedure shown on a sample basis for fitting distributions to 12 months of CPI-U inflation data and one survey round of IESH data, the distribution fitting exercises are performed for each survey round starting from July 2020 to September 2023. For each of these survey rounds, the corresponding 12 months of CPI-U inflation data are fitted with appropriate probability distribution functions. To nowcast the CPI-U of each CPI state under study for the survey month by using the IESH survey data of a single round and the CPI-U inflation data available for the past 12 months (based on the logic that individuals usually poll inflation sentiments based on past experiences), equations (3), (8) and (9) are used. These estimates are named here as ‘1 IESH 12 CPI-U’. Using the same data, forecasting the CPI-U inflation of each CPI state under study for the next three months and for the next year is also done. Chart A4 displays the three-months ahead forecast of CPI-U inflation from October 2020 to December 2023 for each state. To check the robustness of the proposed methodology, the nowcasting and forecasting exercises are then repeated by utilising the following data combinations: i. IESH data pertaining to a single survey round and previous six months’ CPI-U inflation data ‘1 IESH 6 CPI-U’: The logic for such a selection is that individuals usually poll inflation sentiments based on the experiences in the past few months. ii. All IESH data from December 201311 round onwards and CPI-U inflation data of all months from the beginning, i.e., from January 2014 onwards ‘All IESH All CPI-U’: The logic for such a selection is to prevent any loss of information in the survey and the inflation datasets. iii. All IESH data and previous twelve months’ CPI-U inflation data ‘All IESH 12 CPI-U’: This utilises the entire survey data but considers the inflation data from the last year using the logic that individuals usually poll inflation sentiments based on past experiences. iv. All IESH data and previous six months’ CPI-U inflation data ‘All IESH 6 CPI-U’: This utilises the entire survey data but considers the recent inflation data using the logic that individuals usually poll inflation sentiments based on the recent experiences. The CPI-U inflation nowcasts and forecasts are separately derived using the discrete survey data (D) and by adding the estimates of discrete and continuous survey data (D+C). These are then compared with the realised CPI-U12 inflation figures, and the performances of the estimates are gauged by using the error measure Theil’s U given below in equation (10):
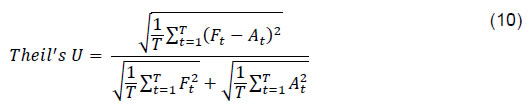
where, Ft is the forecast for time period t, At is the actual at time period t and T is the number of time periods. Lower the value of Theil’s U, the closer is the nowcast/ forecast to the realised figure. The performances of the inflation perceptions (considered as one of the benchmark nowcasts) and expectations (considered as one of the benchmark forecasts) from the IESH data are also measured based on above methodology. Apart from this, another type of nowcasts and forecasts are considered here by removing the bias from the survey estimates as shown in equation (11).

where, ETs is the revised forecast of the Tth month for the sth state derived from the survey estimate, eTs is the inflation perception/ expectation estimate of the Tth month for the sth state from the survey and Ais is the realised official inflation figure of the ith, i = 1,2,…,T, month for the sth state. Furthermore, out-of-sample nowcasts and forecasts based on simple linear regression (named as ‘Reg-based’) of the centre-wise survey-based mean estimates on the corresponding state-wise CPI-U general inflation figures are also taken as benchmark estimates for the comparison purposes based on the performances of nowcasts and forecasts. These estimates are named here as ‘IESH-BA’13. Tables 7(I), 7(II) and 7(III) display the performances of the nowcasts and forecasts14 based on Theil’s U values.
Table 7(I): Performances of Nowcasts: Values of Theil’s U for Nowcasts vis-à-vis Realised Inflation Values |
Data |
GJ |
KT |
MP |
OD |
TN |
DL |
AS |
IESH |
0.275 |
0.242 |
0.151 |
0.241 |
0.238 |
0.418 |
0.266 |
IESH-BA |
0.339 |
0.170 |
0.171 |
0.194 |
0.175 |
0.240 |
0.267 |
Reg-based |
0.197 |
0.088 |
0.156 |
0.182 |
0.152 |
0.176 |
0.208 |
1 IESH 12 CPI-U |
D |
0.125 |
0.111 |
0.115 |
0.163 |
0.111 |
0.198 |
0.199 |
D+C |
0.236 |
0.238 |
0.273 |
0.261 |
0.263 |
0.288 |
0.325 |
1 IESH 6 CPI-U |
D |
0.125 |
0.113 |
0.099 |
0.165 |
0.105 |
0.181 |
0.169 |
D+C |
0.241 |
0.204 |
0.247 |
0.234 |
0.208 |
0.254 |
0.322 |
All IESH All CPI-U |
D |
0.149 |
0.102 |
0.164 |
0.203 |
0.162 |
0.159 |
0.299 |
D+C |
0.161 |
0.187 |
0.212 |
0.188 |
0.189 |
0.272 |
0.233 |
All IESH 12 CPI-U |
D |
0.143 |
0.111 |
0.120 |
0.152 |
0.107 |
0.196 |
0.165 |
D+C |
0.199 |
0.247 |
0.253 |
0.211 |
0.244 |
0.291 |
0.299 |
All IESH 6 CPI-U |
D |
0.138 |
0.110 |
0.110 |
0.165 |
0.105 |
0.182 |
0.158 |
D+C |
0.196 |
0.202 |
0.235 |
0.215 |
0.195 |
0.260 |
0.294 |
|
Data |
TL |
RJ |
JK |
WB |
UP |
MHM |
MHN |
IESH |
0.192 |
0.296 |
0.314 |
0.261 |
0.273 |
0.238 |
0.206 |
IESH-BA |
0.125 |
0.199 |
|
0.223 |
0.111 |
0.141 |
0.130 |
Reg-based |
0.149 |
0.177 |
|
0.204 |
0.114 |
0.155 |
0.164 |
1 IESH 12 CPI-U |
D |
0.115 |
0.145 |
0.108 |
0.152 |
0.116 |
0.129 |
0.116 |
D+C |
0.278 |
0.242 |
0.288 |
0.259 |
0.249 |
0.235 |
0.248 |
1 IESH 6 CPI-U |
D |
0.192 |
0.144 |
0.087 |
0.132 |
0.114 |
0.117 |
0.106 |
D+C |
0.284 |
0.241 |
0.257 |
0.263 |
0.237 |
0.258 |
0.268 |
All IESH All CPI-U |
D |
0.223 |
0.150 |
0.136 |
0.304 |
0.145 |
0.161 |
0.161 |
D+C |
0.222 |
0.204 |
0.222 |
0.225 |
0.180 |
0.218 |
0.220 |
All IESH 12 CPI-U |
D |
0.165 |
0.148 |
0.101 |
0.156 |
0.110 |
0.170 |
0.169 |
D+C |
0.288 |
0.165 |
0.282 |
0.238 |
0.220 |
0.234 |
0.235 |
All IESH 6 CPI-U |
D |
0.177 |
0.154 |
0.091 |
0.149 |
0.132 |
0.121 |
0.122 |
D+C |
0.266 |
0.203 |
0.250 |
0.262 |
0.224 |
0.249 |
0.249 |
|
|
|
|
|
|
|
|
|
Data |
BR |
KL |
CH |
JH |
CHH |
AC |
|
IESH |
0.160 |
0.105 |
0.358 |
0.175 |
0.293 |
0.234 |
|
IESH-BA |
0.225 |
0.385 |
0.144 |
0.172 |
0.220 |
0.100 |
|
Reg-based |
0.201 |
0.106 |
0.132 |
0.163 |
0.190 |
0.113 |
|
1 IESH 12 CPI-U |
D |
0.151 |
0.095 |
0.110 |
0.139 |
0.169 |
0.083 |
|
D+C |
0.271 |
0.289 |
0.259 |
0.310 |
0.300 |
0.215 |
|
1 IESH 6 CPI-U |
D |
0.128 |
0.094 |
0.112 |
0.147 |
0.166 |
0.083 |
|
D+C |
0.269 |
0.258 |
0.243 |
0.298 |
0.282 |
0.194 |
|
All IESH All CPI-U |
D |
0.133 |
0.103 |
0.088 |
0.129 |
0.151 |
0.133 |
|
D+C |
0.207 |
0.202 |
0.221 |
0.261 |
0.237 |
0.140 |
|
All IESH 12 CPI-U |
D |
0.145 |
0.079 |
0.089 |
0.148 |
0.153 |
0.087 |
|
D+C |
0.295 |
0.240 |
0.253 |
0.332 |
0.277 |
0.181 |
|
All IESH 6 CPI-U |
D |
0.124 |
0.141 |
0.084 |
0.135 |
0.154 |
0.094 |
|
D+C |
0.277 |
0.225 |
0.223 |
0.305 |
0.266 |
0.162 |
|
Sources: RBI, MOSPI and Authors’ calculations. |
Table 7(II): Performances of Three-month ahead Forecasts: Values of Theil’s U for Forecasts vis-à-vis Realised Inflation Values |
Data |
GJ |
KT |
MP |
OD |
TN |
DL |
AS |
IESH |
0.322 |
0.298 |
0.207 |
0.297 |
0.308 |
0.462 |
0.322 |
IESH-BA |
0.288 |
0.209 |
0.167 |
0.188 |
0.113 |
0.264 |
0.209 |
Reg-based |
0.172 |
0.101 |
0.156 |
0.186 |
0.122 |
0.189 |
0.171 |
1 IESH 12 CPI-U |
D |
0.145 |
0.127 |
0.122 |
0.164 |
0.115 |
0.244 |
0.225 |
D+C |
0.220 |
0.205 |
0.253 |
0.241 |
0.259 |
0.307 |
0.363 |
1 IESH 6 CPI-U |
D |
0.147 |
0.151 |
0.141 |
0.189 |
0.123 |
0.233 |
0.222 |
D+C |
0.227 |
0.202 |
0.255 |
0.218 |
0.200 |
0.281 |
0.374 |
All IESH All CPI-U |
D |
0.218 |
0.137 |
0.194 |
0.258 |
0.199 |
0.188 |
0.326 |
D+C |
0.181 |
0.173 |
0.219 |
0.212 |
0.184 |
0.264 |
0.266 |
All IESH 12 CPI-U |
D |
0.181 |
0.127 |
0.120 |
0.177 |
0.128 |
0.245 |
0.229 |
D+C |
0.185 |
0.227 |
0.229 |
0.199 |
0.245 |
0.317 |
0.364 |
All IESH 6 CPI-U |
D |
0.195 |
0.157 |
0.134 |
0.209 |
0.141 |
0.230 |
0.249 |
D+C |
0.212 |
0.222 |
0.232 |
0.208 |
0.189 |
0.280 |
0.372 |
|
Data |
TL |
RJ |
JK |
WB |
UP |
MHM |
MHN |
IESH |
0.247 |
0.308 |
0.337 |
0.327 |
0.311 |
0.284 |
0.273 |
IESH-BA |
0.109 |
0.182 |
|
0.149 |
0.101 |
0.106 |
0.077 |
Reg-based |
0.132 |
0.156 |
|
0.156 |
0.097 |
0.133 |
0.134 |
1 IESH 12 CPI-U |
D |
0.133 |
0.169 |
0.165 |
0.171 |
0.142 |
0.163 |
0.151 |
D+C |
0.262 |
0.235 |
0.318 |
0.279 |
0.238 |
0.238 |
0.250 |
1 IESH 6 CPI-U |
D |
0.262 |
0.226 |
0.154 |
0.169 |
0.138 |
0.152 |
0.145 |
D+C |
0.301 |
0.291 |
0.289 |
0.288 |
0.235 |
0.261 |
0.268 |
All IESH All CPI-U |
D |
0.355 |
0.171 |
0.193 |
0.293 |
0.156 |
0.192 |
0.197 |
D+C |
0.312 |
0.232 |
0.262 |
0.199 |
0.185 |
0.222 |
0.222 |
All IESH 12 CPI-U |
D |
0.170 |
0.151 |
0.151 |
0.175 |
0.125 |
0.201 |
0.198 |
D+C |
0.269 |
0.160 |
0.306 |
0.268 |
0.206 |
0.243 |
0.237 |
All IESH 6 CPI-U |
D |
0.285 |
0.185 |
0.149 |
0.179 |
0.154 |
0.156 |
0.163 |
D+C |
0.306 |
0.245 |
0.283 |
0.284 |
0.227 |
0.247 |
0.249 |
|
Data |
BR |
KL |
CH |
JH |
CHH |
AC |
|
IESH |
0.191 |
0.200 |
0.377 |
0.199 |
0.323 |
0.291 |
|
IESH-BA |
0.214 |
0.189 |
0.174 |
0.146 |
0.200 |
0.072 |
|
Reg-based |
0.196 |
0.074 |
0.172 |
0.137 |
0.187 |
0.090 |
|
1 IESH 12 CPI-U |
D |
0.152 |
0.097 |
0.127 |
0.123 |
0.192 |
0.084 |
|
D+C |
0.285 |
0.286 |
0.241 |
0.316 |
0.318 |
0.193 |
|
1 IESH 6 CPI-U |
D |
0.141 |
0.091 |
0.137 |
0.123 |
0.194 |
0.098 |
|
D+C |
0.281 |
0.249 |
0.239 |
0.305 |
0.292 |
0.175 |
|
All IESH All CPI-U |
D |
0.147 |
0.105 |
0.120 |
0.116 |
0.176 |
0.159 |
|
D+C |
0.202 |
0.231 |
0.222 |
0.266 |
0.233 |
0.129 |
|
All IESH 12 CPI-U |
D |
0.151 |
0.090 |
0.097 |
0.131 |
0.179 |
0.101 |
|
D+C |
0.281 |
0.256 |
0.231 |
0.325 |
0.290 |
0.163 |
|
All IESH 6 CPI-U |
D |
0.134 |
0.151 |
0.122 |
0.121 |
0.171 |
0.116 |
|
D+C |
0.274 |
0.247 |
0.225 |
0.306 |
0.266 |
0.146 |
|
Sources: RBI, MOSPI and Authors’ calculations. |
Table 7(III): Performances of One-year ahead Forecasts: Values of Theil’s U for Forecasts vis-à-vis Realised Inflation Values |
Data |
GJ |
KT |
MP |
OD |
TN |
DL |
AS |
IESH |
0.323 |
0.296 |
0.207 |
0.273 |
0.319 |
0.441 |
0.344 |
IESH-BA |
0.460 |
0.165 |
0.283 |
0.407 |
0.211 |
0.274 |
0.627 |
Reg-based |
0.156 |
0.095 |
0.156 |
0.174 |
0.131 |
0.196 |
0.143 |
1 IESH 12 CPI-U |
D |
0.163 |
0.142 |
0.134 |
0.136 |
0.150 |
0.282 |
0.286 |
D+C |
0.213 |
0.206 |
0.254 |
0.245 |
0.262 |
0.331 |
0.432 |
1 IESH 6 CPI-U |
D |
0.152 |
0.138 |
0.156 |
0.115 |
0.136 |
0.304 |
0.298 |
D+C |
0.234 |
0.167 |
0.237 |
0.183 |
0.151 |
0.335 |
0.454 |
All IESH All CPI-U |
D |
0.249 |
0.128 |
0.213 |
0.227 |
0.220 |
0.221 |
0.282 |
D+C |
0.153 |
0.137 |
0.175 |
0.165 |
0.094 |
0.278 |
0.201 |
All IESH 12 CPI-U |
D |
0.222 |
0.130 |
0.155 |
0.174 |
0.180 |
0.304 |
0.276 |
D+C |
0.214 |
0.235 |
0.244 |
0.224 |
0.259 |
0.351 |
0.411 |
All IESH 6 CPI-U |
D |
0.202 |
0.150 |
0.166 |
0.178 |
0.187 |
0.323 |
0.302 |
D+C |
0.234 |
0.195 |
0.212 |
0.184 |
0.158 |
0.350 |
0.444 |
|
Data |
TL |
RJ |
JK |
WB |
UP |
MHM |
MHN |
IESH |
0.246 |
0.276 |
0.416 |
0.328 |
0.289 |
0.243 |
0.277 |
IESH-BA |
0.168 |
0.362 |
|
0.284 |
0.210 |
0.318 |
0.255 |
Reg-based |
0.123 |
0.177 |
|
0.122 |
0.130 |
0.167 |
0.165 |
1 IESH 12 CPI-U |
D |
0.113 |
0.152 |
0.107 |
0.203 |
0.146 |
0.167 |
0.126 |
D+C |
0.205 |
0.200 |
0.318 |
0.283 |
0.227 |
0.222 |
0.223 |
1 IESH 6 CPI-U |
D |
0.238 |
0.155 |
0.126 |
0.213 |
0.176 |
0.186 |
0.148 |
D+C |
0.257 |
0.231 |
0.310 |
0.333 |
0.267 |
0.255 |
0.271 |
All IESH All CPI-U |
D |
0.390 |
0.176 |
0.201 |
0.318 |
0.207 |
0.238 |
0.243 |
D+C |
0.299 |
0.193 |
0.273 |
0.228 |
0.203 |
0.234 |
0.231 |
All IESH 12 CPI-U |
D |
0.189 |
0.196 |
0.107 |
0.215 |
0.148 |
0.211 |
0.219 |
D+C |
0.208 |
0.176 |
0.305 |
0.278 |
0.186 |
0.230 |
0.233 |
All IESH 6 CPI-U |
D |
0.297 |
0.209 |
0.141 |
0.223 |
0.184 |
0.178 |
0.185 |
|
D+C |
0.290 |
0.221 |
0.307 |
0.324 |
0.248 |
0.257 |
0.262 |
|
Data |
BR |
KL |
CH |
JH |
CHH |
AC |
|
IESH |
0.202 |
0.266 |
0.341 |
0.191 |
0.323 |
0.283 |
|
IESH-BA |
0.483 |
0.382 |
0.188 |
0.283 |
0.185 |
0.213 |
|
Reg-based |
0.191 |
0.073 |
0.118 |
0.118 |
0.158 |
0.102 |
|
1 IESH 12 CPI-U |
D |
0.198 |
0.098 |
0.156 |
0.159 |
0.211 |
0.097 |
|
D+C |
0.277 |
0.268 |
0.301 |
0.329 |
0.322 |
0.176 |
|
1 IESH 6 CPI-U |
D |
0.166 |
0.090 |
0.147 |
0.170 |
0.184 |
0.104 |
|
D+C |
0.275 |
0.230 |
0.245 |
0.318 |
0.301 |
0.175 |
|
All IESH All CPI-U |
D |
0.179 |
0.139 |
0.120 |
0.146 |
0.156 |
0.187 |
|
D+C |
0.202 |
0.189 |
0.175 |
0.281 |
0.240 |
0.117 |
|
All IESH 12 CPI-U |
D |
0.196 |
0.118 |
0.139 |
0.159 |
0.200 |
0.131 |
|
D+C |
0.283 |
0.224 |
0.280 |
0.341 |
0.294 |
0.151 |
|
All IESH 6 CPI-U |
D |
0.172 |
0.167 |
0.159 |
0.155 |
0.166 |
0.132 |
|
D+C |
0.269 |
0.239 |
0.236 |
0.319 |
0.279 |
0.140 |
|
Sources: RBI, MOSPI and Authors’ calculations. |
From the tables, adjustment of bias using historical deviations of inflation perceptions and expectations from the realised inflation in the ‘IESH-BA’ estimates do not produce nowcasts and forecasts that are closer to the realised inflation than the nowcasts and forecasts obtained using this methodology for most states. The estimates from discrete estimates bear lower errors in nowcasts and forecasts as compared to the sum of discrete and continuous estimates. Table 8 displays the methods for which the error measure Theil’s U is lower than the errors in the remaining methods. For the states excluding Karnataka, Assam, West Bengal, Uttar Pradesh and Kerala, the proposed exercise yields better inflation nowcasts and forecasts than the other benchmark methods.
Table 8: Methods Producing Estimates with Errors Lower than Other Methods |
State |
Nowcasts |
Three-months-ahead Forecasts |
One-Year-Ahead Forecasts |
Gujarat |
1 IESH 6 CPI-U |
1 IESH 12 CPI-U |
1 IESH 6 CPI-U |
Karnataka |
Reg-based |
Reg-based |
Reg-based |
Madhya Pradesh |
1 IESH 6 CPI-U |
All IESH 12 CPI-U |
1 IESH 12 CPI-U |
Odisha |
All IESH 12 CPI-U |
1 IESH 12 CPI-U |
1 IESH 6 CPI-U |
Tamil Nadu |
All IESH 6 CPI-U |
IESH-BA |
All IESH All CPI-U |
Delhi |
All IESH All CPI-U |
All IESH All CPI-U |
Reg-based |
Assam |
All IESH 6 CPI-U |
Reg-based |
Reg-based |
Telangana |
1 IESH 12 CPI-U |
IESH-BA |
1 IESH 12 CPI-U |
Rajasthan |
1 IESH 6 CPI-U |
All IESH 12 CPI-U |
1 IESH 12 CPI-U |
Jammu and Kashmir |
1 IESH 6 CPI-U |
All IESH 6 CPI-U |
1 IESH 12 CPI-U |
West Bengal |
1 IESH 6 CPI-U |
IESH-BA |
Reg-based |
Uttar Pradesh |
All IESH 12 CPI-U |
Reg-based |
Reg-based |
Maharashtra (using Mumbai) |
1 IESH 6 CPI-U |
IESH-BA |
1 IESH 12 CPI-U |
Maharashtra (using Nagpur) |
1 IESH 6 CPI-U |
IESH-BA |
1 IESH 12 CPI-U |
Bihar |
All IESH 6 CPI-U |
All IESH 6 CPI-U |
1 IESH 6 CPI-U |
Kerala |
All IESH 12 CPI-U |
Reg-based |
Reg-based |
Chandigarh |
All IESH 6 CPI-U |
All IESH 12 CPI-U |
Reg-based |
Jharkhand |
All IESH All CPI-U |
All IESH All CPI-U |
Reg-based |
Chhattisgarh |
All IESH All CPI-U |
All IESH 6 CPI-U |
All IESH All CPI-U |
All India |
1 IESH 6 CPI-U |
IESH-BA |
1 IESH 12 CPI-U |
Source: Authors’ calculations. |
Tables A4, A4 (I), 4(II), A5, A5 (I), A5 (II), A6, A6 (I) and A6 (II) display the performances of the nowcasts and forecasts, computed using other measures of errors defined in Table A3. An alternative comparison of the forecasts is made in terms of the percentage number of times the directions of forecasts match with the directions of the realised inflation figures from one period to another. The results are shown in Tables A7, A7 (I), and A7 (II). In about half of the states, the nowcasts using the proposed methodology display more directional matches with the realised inflation than the nowcasts from IESH, ‘IESH-BA’ and ‘Reg-based’. The three-months-ahead and one-year-ahead forecasts obtained using the proposed methodology in most of the states display more directional matches with the realised inflation than the forecasts derived from IESH, ‘IESH-BA’ and ‘Reg-based’. This phenomenon is more noticeable in case of the one-year ahead forecasts. Further, in case of three-month ahead and one-year ahead forecasts, the directional matches of proposed estimates and realised inflation are more compared to the directional matches of the estimates from IESH, ‘IESH-BA’ and ‘Reg-based’ with the direction of the realised inflation. Part of the forecast errors arise because the estimates from the proposed methodologies (explored here), obtained using the survey data of the cities/ centres (where IESH are being conducted), are assumed to be the forecasts of the CPI-U inflation of the entire state. Further, the out-of-sample nowcasts and forecasts are studied for (a) the COVID-19 pandemic period and (b) the post-pandemic period, during which economic uncertainty was a major challenge in forecasting macroeconomic variables. The one-quarter ahead and one-year ahead CPI – Combined (CPI-C) inflation forecasts (for the study period) of the professional forecasters from the Survey of Professional Forecasters conducted by the RBI on a bimonthly basis are plotted against the realised CPI-C inflation in Chart A5. The errors in one-quarter ahead and one-year ahead CPI-C inflation forecasts of the professional forecasters are compared with the error measures (the least error measure obtained among the five proposed measures) of the forecasts pertaining to all states combined, i.e., ‘AC’, in the Tables A4, A4 (I), A4 (II), A5, A5 (I), A5 (II), A6, A6 (I), and A6 (II), and presented in Table A8. It is observed that the error measures are quite competitive for the three-month ahead forecasts and lower in quantum for the one-year ahead forecasts obtained from the proposed methodologies. The findings indicate that the proposed method emerges with better performance than a few benchmarks for most of the states. Thus, it opens a path for further exploration in comparison with the conventional econometric model-based forecasts. V. Conclusion The modelling of inflation expectations to map these data with the realised inflation prints is an evolving area of research. In this paper, we propose a new approach to modelling inflation expectations, which not only uses centre-wise survey data and state-wise inflation data but also redistributes the inflation expectation of respondents suitably to gain further precision. For most of the states, the results show a noticeable reduction in the quantum of nowcast/ forecast errors in state-level nowcasts/ forecasts obtained using the proposed methodology when compared with the errors of the survey forecasts, bias-adjusted survey forecasts and linear regression-based forecasts. The percentage of occurrences of directional matches of the nowcasts/ forecasts and the realised inflation figures are more in the case of nowcasts, three-months-ahead forecasts and especially one-year-ahead forecasts obtained using our method than in the estimates ‘IESH’, ‘IESH-BA’ and ‘Reg-based’. Variation in state-wise inflation can be attributed to factors like transport costs (like fuel prices, tolls, etc.), differences in the state taxation policies and supply chain efficiencies. In such a scenario especially in an inflation targeting regime, one of the plausible ways to reduce forecast error would be to aggregate state-level forecasts, as attempted in this paper. Further, studying these forecasts in comparison with complex econometric model-based state-level forecasts may throw more light on the performance of the proposed method. Going forward, it is possible to develop a model as a mixture of the existing approaches, viz., econometric modelling, Bayesian forecasting, and the approach proposed in this paper to gain greater precision in forecasting inflation.
References Abildgren, K., & Kuchler, A. (2021). Revisiting the inflation perception conundrum. Journal of Macroeconomics, 67, https://doi.org/10.1016/j.jmacro.2020.103264 Banerjee, N., & Das, A. (2011). Fan chart: methodology and its application to inflation forecasting in India. RBI Working Paper Series, WPS (DEPR): 5/2011. Binder, C. (2017). Measuring uncertainty based on rounding: new method and application to inflation expectations. Journal of Monetary Economics, 90, 1-12. Blix, M., & Sellin, P. (1998). Uncertainty bands for inflation forecasts. Sveriges Riksbank Working Paper Series, No. 65. Ciumara, R. (2006). An actuarial model based on the composite Weibull-Pareto distribution. Mathematical Reports, 8(58), No. 4/2006. Cooray, K., & Ananda, M. M. A. (2005). Modelling actuarial data with a composite Lognormal-Pareto model. Scandinavian Actuarial Journal, (5), 321-334. Fama, E. F. (1965). The behaviour of stock market prices. Journal of Business, 38, 34-105. Frigessi, A., Haug, O., & Rue, A. (2002). Dynamic mixture model for unsupervised tail estimation without threshold selection. Extremes, 5, 219-235. Goldfayn-Frank, O., & Wohlfart, J. (2020). Expectation formation in a new environment: evidence from the German reunification. Journal of Monetary Economics, 115, 301-320. Goyal, A., & Parab, P. (2019). Inflation convergence and anchoring of expectations in India. Working Paper, No. 023, Indira Gandhi Institute of Development Research, July. Johnson, N. L., Kotz, S., & Balakrishnan, N. (1994). Continuous Univariate Distributions, Vol. 1, 2nd ed. Wiley, New York. Jonung, L. (1981). Perceived and expected rates of inflation in Sweden. The American Economic Review, 71(5), 961-968. Krifka, M. (2009). Approximate interpretations of number words: a case for strategic communication. In Erhard W. H., & John A. N. (Eds.), Theory and evidence in semantics (pp. 109-132). Standford, CSLI Publications. Moore, A. B. (1964). Some characteristics of changes in common stock prices. In Cootner, P. (Eds.), The random character of stock market prices. M.I.T. Press, Cambridge, Mass. Malmendier, U., & Nagel, S. (2016). Learning from inflation experiences. The Quarterly Journal of Economics, 131(1), 53-87. Nadarajah, S., & Bakar, S. A. A. (2014). New composite models for the Danish fire insurance data. Scandinavian Actuarial Journal, 2, 180-187. Osborne, M. (1959). Brownian motion in the stock market. Operations Research, 7, 145-173. Praetz, P. (1969). Australian share prices and the random walk hypothesis. Australian Journal of Statistics, 11, 123-139. Praetz, P. (1972). The Distribution of share price changes. The Journal of Business, 45, 49-55. Qiao, C., Myers, A. T., & Natarajan, A. (2022). Probability distribution of time series with temporal correlation: from frequently occurring to extreme values. Ocean Engineering, 248, 1-19. RBI. (2010). Inflation expectations survey of households. Retrieved April 19, 2010 from https://www.rbi.org.in/Scripts/PublicationsView.aspx?id=14036 Reiche, L., & Meyler, A. (2022). Making sense of consumer inflation expectations: the role of uncertainty. Discussion Paper, No. 159, European Economy Discussion Papers, European Commission, February. Scollnik, D. P. (2007). On composite Lognormal-Pareto model. Scandinavian Actuarial Journal, 2007(1), 20-33. Scollnik, D. P., & Sun, C. (2012). Modelling with Weibull-Pareto models. North American Actuarial Journal, 16 (2), 260-272. Shaw, P. (2024). Reading consumers’ mind: an analysis of inflation expectations. Macroeconomics and Finance in Emerging Market Economies, https://doi.org/10.1080/17520843.2024.2352955 Singh, D. P., Mishra, A., & Shaw, P. (2024). Taking cognisance of households’ inflation expectations in India. Macroeconomics and Finance in Emerging Market Economies, https://doi.org/10.1080/17520843.2024.2350250 Sinha, R. K. (2023). Inflation and inflation expectations: a distributional mapping. Monthly Bulletin, Reserve Bank of India, September. Souleles, N. C. (2004). Expectations, heterogeneous forecast errors, and consumption: micro evidence from the Michigen consumer sentiment surveys. Journal of Money, Credit and Banking, 36(1), 39-72.
Annex
Table A2: Fitting of Poisson(λ) Distribution to Discrete Survey Data of September 2023 Round: Estimated Values of λ |
Survey Centre |
Inflation Perceptions |
Three-months-ahead Inflation Expectations |
One-year-ahead Inflation Expectations |
Ahmedabad |
0.054 |
0.017 |
0.169 |
Bangalore |
0.114 |
0.301 |
0.460 |
Bhopal |
0.034 |
0.042 |
0.224 |
Bhubaneswar |
0.091 |
0.357 |
0.316 |
Chennai |
0.092 |
0.190 |
0.250 |
Delhi |
0.425 |
0.619 |
0.692 |
Guwahati |
0.067 |
0.077 |
0.043 |
Hyderabad |
0.078 |
0.276 |
0.312 |
Jaipur |
0.045 |
0.100 |
0.091 |
Jammu |
0.174 |
0.531 |
0.333 |
Kolkata |
0.318 |
0.616 |
0.662 |
Lucknow |
0.230 |
0.333 |
0.255 |
Mumbai |
0.257 |
0.264 |
0.159 |
Nagpur |
0.205 |
0.117 |
0.483 |
Patna |
0.300 |
0.300 |
0.455 |
Thiruvananthapuram |
0.455 |
0.125 |
0.182 |
Chandigarh |
0.474 |
0.105 |
0.481 |
Ranchi |
0.429 |
0.333 |
0.333 |
Raipur |
0.167 |
0.161 |
0.258 |
Sources: RBI and Authors’ calculations. |
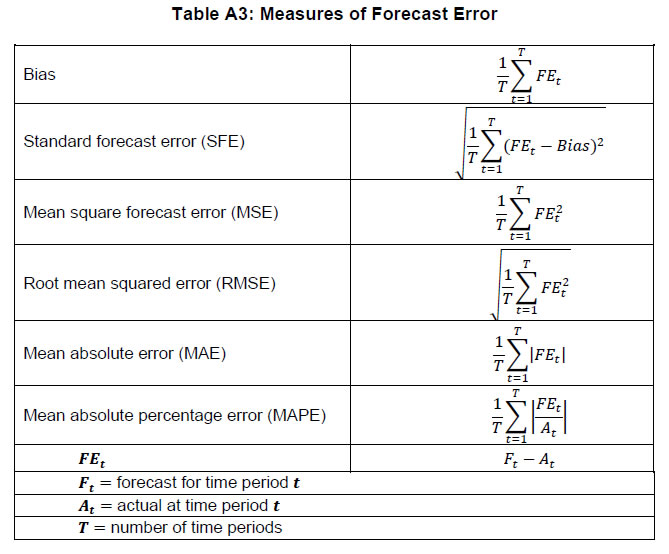
Table A4: Performances of Nowcasts: Values of Errors for Nowcasts vis-à-vis Realised Inflation Values |
Data |
|
Measures of Forecast Error |
GJ |
KT |
MP |
OD |
TN |
DL |
AS |
IESH |
|
Bias |
3.877 |
3.585 |
2.023 |
3.088 |
3.594 |
5.929 |
3.579 |
MSE |
17.970 |
13.892 |
5.472 |
12.662 |
15.327 |
37.463 |
16.270 |
RMSE |
4.239 |
3.727 |
2.339 |
3.558 |
3.915 |
6.121 |
4.034 |
MAE |
4.506 |
4.039 |
2.197 |
3.661 |
4.118 |
7.308 |
4.150 |
MAPE |
0.779 |
0.658 |
0.351 |
0.678 |
0.645 |
1.872 |
0.838 |
SFE |
1.756 |
1.046 |
1.203 |
1.812 |
1.590 |
1.558 |
1.907 |
IESH-BA |
|
Bias |
-2.55 |
2.15 |
-1.65 |
-1.07 |
-1.30 |
2.11 |
-1.91 |
MSE |
9.454 |
5.665 |
4.091 |
4.277 |
4.096 |
6.748 |
7.094 |
RMSE |
3.075 |
2.380 |
2.023 |
2.068 |
2.024 |
2.598 |
2.663 |
MAE |
2.981 |
2.351 |
1.860 |
1.740 |
1.764 |
2.355 |
2.566 |
MAPE |
0.443 |
0.409 |
0.253 |
0.280 |
0.241 |
0.781 |
0.440 |
SFE |
1.756 |
1.046 |
1.203 |
1.812 |
1.590 |
1.558 |
1.907 |
Reg-based |
|
Bias |
-1.518 |
0.535 |
-1.424 |
-1.042 |
-1.029 |
0.413 |
0.020 |
MSE |
3.855 |
1.178 |
3.524 |
3.707 |
3.245 |
2.572 |
5.569 |
RMSE |
1.963 |
1.085 |
1.877 |
1.925 |
1.801 |
1.604 |
2.360 |
MAE |
1.754 |
0.846 |
1.693 |
1.539 |
1.556 |
1.419 |
1.852 |
MAPE |
0.265 |
0.165 |
0.229 |
0.230 |
0.218 |
0.472 |
0.347 |
SFE |
1.276 |
0.968 |
1.254 |
1.659 |
1.515 |
1.588 |
2.418 |
1 IESH 12 CPI-U |
D |
Bias |
0.062 |
-0.065 |
0.539 |
0.820 |
0.411 |
-0.074 |
0.889 |
MSE |
2.128 |
1.706 |
2.616 |
4.138 |
2.188 |
2.990 |
6.316 |
RMSE |
1.459 |
1.306 |
1.618 |
2.034 |
1.479 |
1.729 |
2.513 |
MAE |
1.261 |
1.054 |
1.287 |
1.805 |
1.282 |
1.426 |
2.105 |
MAPE |
0.236 |
0.188 |
0.220 |
0.355 |
0.226 |
0.405 |
0.470 |
SFE |
1.494 |
1.337 |
1.563 |
1.908 |
1.456 |
1.770 |
2.409 |
D+C |
Bias |
2.243 |
2.120 |
4.119 |
3.480 |
3.625 |
1.773 |
3.703 |
MSE |
11.166 |
11.491 |
23.464 |
15.805 |
19.398 |
9.805 |
27.005 |
RMSE |
3.342 |
3.390 |
4.844 |
3.976 |
4.404 |
3.131 |
5.197 |
MAE |
3.020 |
3.201 |
5.207 |
4.130 |
4.521 |
2.761 |
5.263 |
MAPE |
0.507 |
0.529 |
0.732 |
0.737 |
0.682 |
0.769 |
0.938 |
SFE |
2.538 |
2.711 |
2.612 |
1.971 |
2.563 |
2.645 |
3.736 |
1 IESH 6 CPI-U |
D |
Bias |
0.027 |
-0.435 |
0.237 |
0.439 |
0.210 |
-0.169 |
0.332 |
MSE |
2.111 |
1.654 |
1.868 |
4.031 |
1.908 |
2.472 |
4.239 |
RMSE |
1.453 |
1.286 |
1.367 |
2.008 |
1.381 |
1.572 |
2.059 |
MAE |
1.302 |
1.100 |
1.133 |
1.694 |
1.089 |
1.228 |
1.769 |
MAPE |
0.240 |
0.187 |
0.197 |
0.314 |
0.192 |
0.337 |
0.381 |
SFE |
1.488 |
1.240 |
1.379 |
2.008 |
1.399 |
1.602 |
2.082 |
D+C |
Bias |
2.160 |
1.160 |
3.057 |
2.193 |
1.943 |
1.198 |
3.098 |
MSE |
11.497 |
7.396 |
17.376 |
10.765 |
9.798 |
7.062 |
25.948 |
RMSE |
3.391 |
2.720 |
4.168 |
3.281 |
3.130 |
2.657 |
5.094 |
MAE |
3.064 |
2.491 |
4.020 |
3.120 |
2.729 |
2.225 |
5.046 |
MAPE |
0.515 |
0.409 |
0.569 |
0.582 |
0.445 |
0.570 |
0.831 |
SFE |
2.678 |
2.520 |
2.904 |
2.500 |
2.515 |
2.430 |
4.143 |
All IESH All CPI-U |
D |
Bias |
-0.946 |
-0.516 |
-1.220 |
-1.103 |
-1.108 |
0.222 |
-1.785 |
MSE |
2.512 |
1.320 |
4.040 |
4.577 |
3.618 |
2.054 |
8.333 |
RMSE |
1.585 |
1.149 |
2.010 |
2.139 |
1.902 |
1.433 |
2.887 |
MAE |
1.446 |
0.966 |
1.917 |
1.780 |
1.718 |
1.200 |
2.420 |
MAPE |
0.237 |
0.155 |
0.276 |
0.276 |
0.249 |
0.368 |
0.348 |
SFE |
1.303 |
1.052 |
1.637 |
1.878 |
1.584 |
1.451 |
2.325 |
D+C |
Bias |
0.981 |
1.871 |
1.211 |
0.664 |
1.275 |
2.248 |
-0.637 |
MSE |
4.146 |
6.680 |
10.146 |
5.486 |
7.319 |
9.542 |
6.532 |
RMSE |
2.036 |
2.585 |
3.185 |
2.342 |
2.705 |
3.089 |
2.556 |
MAE |
1.588 |
2.433 |
2.624 |
1.918 |
2.212 |
2.857 |
2.378 |
MAPE |
0.284 |
0.406 |
0.410 |
0.382 |
0.379 |
0.754 |
0.456 |
SFE |
1.828 |
1.828 |
3.019 |
2.302 |
2.445 |
2.171 |
2.536 |
All IESH 12 CPI-U |
D |
Bias |
-0.659 |
-0.226 |
-0.124 |
-0.101 |
-0.238 |
-0.152 |
-0.001 |
MSE |
2.442 |
1.666 |
2.567 |
3.083 |
1.833 |
2.890 |
3.775 |
RMSE |
1.563 |
1.291 |
1.602 |
1.756 |
1.354 |
1.700 |
1.943 |
MAE |
1.375 |
1.008 |
1.321 |
1.408 |
1.187 |
1.436 |
1.701 |
MAPE |
0.233 |
0.175 |
0.214 |
0.255 |
0.195 |
0.405 |
0.359 |
SFE |
1.452 |
1.302 |
1.637 |
1.796 |
1.366 |
1.735 |
1.991 |
D+C |
Bias |
1.317 |
2.400 |
3.338 |
2.399 |
2.994 |
1.790 |
2.183 |
MSE |
6.926 |
12.880 |
18.657 |
8.883 |
15.553 |
10.069 |
19.603 |
RMSE |
2.632 |
3.589 |
4.319 |
2.980 |
3.944 |
3.173 |
4.427 |
MAE |
2.316 |
3.608 |
4.494 |
2.949 |
3.935 |
2.796 |
4.101 |
MAPE |
0.409 |
0.592 |
0.640 |
0.552 |
0.597 |
0.780 |
0.689 |
SFE |
2.335 |
2.734 |
2.809 |
1.813 |
2.630 |
2.685 |
3.947 |
All IESH 6 CPI-U |
D |
Bias |
-0.651 |
-0.661 |
-0.501 |
-0.443 |
-0.528 |
-0.236 |
-0.335 |
MSE |
2.279 |
1.511 |
2.062 |
3.439 |
1.683 |
2.465 |
3.331 |
RMSE |
1.510 |
1.229 |
1.436 |
1.854 |
1.297 |
1.570 |
1.825 |
MAE |
1.335 |
0.996 |
1.275 |
1.431 |
1.126 |
1.293 |
1.509 |
MAPE |
0.222 |
0.159 |
0.202 |
0.243 |
0.179 |
0.362 |
0.299 |
SFE |
1.396 |
1.062 |
1.379 |
1.845 |
1.214 |
1.590 |
1.838 |
D+C |
Bias |
1.023 |
1.319 |
2.314 |
1.056 |
1.233 |
1.343 |
1.709 |
MSE |
6.336 |
7.498 |
14.550 |
7.763 |
7.856 |
7.673 |
18.683 |
RMSE |
2.517 |
2.738 |
3.814 |
2.786 |
2.803 |
2.770 |
4.322 |
MAE |
2.178 |
2.415 |
3.579 |
2.461 |
2.365 |
2.359 |
3.881 |
MAPE |
0.378 |
0.386 |
0.512 |
0.455 |
0.396 |
0.601 |
0.605 |
SFE |
2.357 |
2.459 |
3.107 |
2.642 |
2.580 |
2.483 |
4.068 |
Sources: RBI, MOSPI and Authors’ calculations. |
Table A4 (I): Performances of Nowcasts: Values of Errors for Nowcasts vis-à-vis Realised Inflation Values (contd.) |
Data |
Measures of Forecast Error |
TL |
RJ |
JK |
WB |
UP |
MHM |
MHN |
IESH |
Bias |
3.075 |
3.971 |
1.999 |
4.123 |
4.204 |
3.680 |
3.045 |
MSE |
11.440 |
20.730 |
26.044 |
21.045 |
19.383 |
15.532 |
10.825 |
RMSE |
3.382 |
4.553 |
5.103 |
4.587 |
4.403 |
3.941 |
3.290 |
MAE |
3.452 |
4.864 |
5.631 |
4.879 |
4.873 |
4.207 |
3.394 |
MAPE |
0.463 |
0.937 |
0.814 |
0.815 |
0.780 |
0.646 |
0.527 |
SFE |
1.444 |
2.283 |
4.811 |
2.062 |
1.340 |
1.445 |
1.278 |
IESH-BA |
Bias |
-0.96 |
-0.22 |
|
-1.68 |
-0.10 |
-0.93 |
-0.94 |
MSE |
2.908 |
5.012 |
|
6.880 |
1.719 |
2.860 |
2.431 |
RMSE |
1.705 |
2.239 |
|
2.623 |
1.311 |
1.691 |
1.559 |
MAE |
1.476 |
1.932 |
|
2.291 |
1.011 |
1.546 |
1.360 |
MAPE |
0.190 |
0.373 |
|
0.291 |
0.178 |
0.236 |
0.203 |
SFE |
1.444 |
2.283 |
|
2.062 |
1.340 |
1.445 |
1.278 |
Reg-based |
Bias |
-1.537 |
-0.645 |
|
-1.203 |
-0.676 |
-1.263 |
-1.452 |
MSE |
3.728 |
3.606 |
|
6.215 |
1.643 |
3.244 |
3.512 |
RMSE |
1.931 |
1.899 |
|
2.493 |
1.282 |
1.801 |
1.874 |
MAE |
1.691 |
1.583 |
|
2.079 |
1.097 |
1.609 |
1.685 |
MAPE |
0.201 |
0.284 |
|
0.275 |
0.182 |
0.227 |
0.232 |
SFE |
1.197 |
1.830 |
|
2.238 |
1.116 |
1.316 |
1.215 |
1 IESH 12 CPI-U |
D |
Bias |
0.037 |
0.616 |
0.410 |
0.037 |
0.209 |
-0.450 |
0.423 |
MSE |
2.810 |
3.105 |
2.153 |
4.274 |
1.993 |
2.584 |
2.404 |
RMSE |
1.676 |
1.762 |
1.467 |
2.067 |
1.412 |
1.607 |
1.551 |
MAE |
1.301 |
1.621 |
1.210 |
1.835 |
1.124 |
1.419 |
1.357 |
MAPE |
0.187 |
0.325 |
0.214 |
0.290 |
0.213 |
0.219 |
0.228 |
SFE |
1.717 |
1.692 |
1.444 |
2.118 |
1.431 |
1.581 |
1.529 |
D+C |
Bias |
3.761 |
3.003 |
4.125 |
3.162 |
2.771 |
2.448 |
3.247 |
MSE |
26.768 |
12.522 |
25.917 |
19.243 |
13.757 |
13.617 |
16.424 |
RMSE |
5.174 |
3.539 |
5.091 |
4.387 |
3.709 |
3.690 |
4.053 |
MAE |
5.276 |
3.441 |
5.555 |
4.411 |
3.676 |
3.413 |
4.057 |
MAPE |
0.663 |
0.650 |
0.788 |
0.666 |
0.617 |
0.502 |
0.596 |
SFE |
3.641 |
1.917 |
3.057 |
3.116 |
2.527 |
2.829 |
2.485 |
1 IESH 6 CPI-U |
D |
Bias |
-0.827 |
0.369 |
0.057 |
-0.005 |
-0.097 |
-0.456 |
0.253 |
MSE |
7.207 |
2.972 |
1.351 |
3.281 |
1.830 |
2.151 |
1.964 |
RMSE |
2.685 |
1.724 |
1.162 |
1.811 |
1.353 |
1.466 |
1.401 |
MAE |
2.251 |
1.449 |
0.880 |
1.545 |
1.120 |
1.277 |
1.267 |
MAPE |
0.292 |
0.264 |
0.150 |
0.240 |
0.205 |
0.194 |
0.210 |
SFE |
2.617 |
1.725 |
1.190 |
1.856 |
1.383 |
1.428 |
1.412 |
D+C |
Bias |
1.626 |
2.556 |
3.129 |
3.040 |
1.932 |
2.991 |
3.431 |
MSE |
23.426 |
11.922 |
18.763 |
20.798 |
11.423 |
17.672 |
19.928 |
RMSE |
4.840 |
3.453 |
4.332 |
4.560 |
3.380 |
4.204 |
4.464 |
MAE |
5.129 |
3.223 |
4.247 |
4.292 |
3.172 |
4.118 |
4.408 |
MAPE |
0.652 |
0.553 |
0.581 |
0.569 |
0.519 |
0.591 |
0.631 |
SFE |
4.671 |
2.378 |
3.070 |
3.483 |
2.842 |
3.026 |
2.927 |
All IESH All CPI-U |
D |
Bias |
-2.135 |
-0.557 |
-0.694 |
-2.389 |
-1.097 |
-1.224 |
-1.185 |
MSE |
7.878 |
2.649 |
2.930 |
11.570 |
2.506 |
3.613 |
3.642 |
RMSE |
2.807 |
1.628 |
1.712 |
3.401 |
1.583 |
1.901 |
1.908 |
MAE |
2.670 |
1.306 |
1.461 |
2.938 |
1.369 |
1.747 |
1.754 |
MAPE |
0.333 |
0.243 |
0.221 |
0.330 |
0.215 |
0.252 |
0.254 |
SFE |
1.866 |
1.567 |
1.603 |
2.481 |
1.169 |
1.490 |
1.533 |
D+C |
Bias |
-0.078 |
1.801 |
1.731 |
-0.906 |
0.785 |
2.141 |
2.044 |
MSE |
11.282 |
7.481 |
11.654 |
8.364 |
5.451 |
11.245 |
11.354 |
RMSE |
3.359 |
2.735 |
3.414 |
2.892 |
2.335 |
3.353 |
3.370 |
MAE |
2.872 |
2.596 |
3.151 |
2.422 |
1.969 |
2.827 |
2.840 |
MAPE |
0.354 |
0.533 |
0.473 |
0.305 |
0.327 |
0.413 |
0.415 |
SFE |
3.441 |
2.110 |
3.015 |
2.814 |
2.253 |
2.645 |
2.745 |
All IESH 12 CPI-U |
D |
Bias |
-0.677 |
-0.480 |
0.057 |
-0.433 |
-0.431 |
-0.902 |
-0.826 |
MSE |
5.295 |
2.620 |
1.794 |
4.196 |
1.601 |
4.278 |
4.255 |
RMSE |
2.301 |
1.618 |
1.340 |
2.049 |
1.265 |
2.068 |
2.063 |
MAE |
1.734 |
1.312 |
1.133 |
1.792 |
1.007 |
1.722 |
1.726 |
MAPE |
0.221 |
0.241 |
0.197 |
0.272 |
0.181 |
0.253 |
0.257 |
SFE |
2.254 |
1.584 |
1.371 |
2.052 |
1.219 |
1.907 |
1.937 |
D+C |
Bias |
3.336 |
1.356 |
3.705 |
2.680 |
2.078 |
1.819 |
1.866 |
MSE |
27.952 |
4.520 |
23.901 |
15.368 |
9.752 |
12.632 |
12.841 |
RMSE |
5.287 |
2.126 |
4.889 |
3.920 |
3.123 |
3.554 |
3.583 |
MAE |
5.689 |
1.598 |
5.332 |
3.781 |
3.110 |
3.366 |
3.435 |
MAPE |
0.718 |
0.359 |
0.758 |
0.579 |
0.535 |
0.514 |
0.523 |
SFE |
4.203 |
1.678 |
3.268 |
2.931 |
2.389 |
3.129 |
3.135 |
All IESH 6 CPI-U |
D |
Bias |
-1.227 |
-0.757 |
-0.203 |
-0.518 |
-0.684 |
-0.532 |
-0.520 |
MSE |
5.619 |
2.710 |
1.408 |
3.888 |
2.230 |
2.242 |
2.314 |
RMSE |
2.370 |
1.646 |
1.186 |
1.972 |
1.493 |
1.497 |
1.521 |
MAE |
1.973 |
1.280 |
1.038 |
1.664 |
1.196 |
1.308 |
1.340 |
MAPE |
0.245 |
0.211 |
0.171 |
0.242 |
0.209 |
0.199 |
0.205 |
SFE |
2.078 |
1.498 |
1.198 |
1.950 |
1.360 |
1.434 |
1.465 |
D+C |
Bias |
1.373 |
1.147 |
2.769 |
2.487 |
1.321 |
2.550 |
2.394 |
MSE |
19.576 |
6.932 |
16.957 |
19.699 |
9.392 |
15.522 |
15.391 |
RMSE |
4.425 |
2.633 |
4.118 |
4.438 |
3.065 |
3.940 |
3.923 |
MAE |
4.557 |
2.157 |
4.134 |
3.976 |
2.824 |
3.713 |
3.664 |
MAPE |
0.585 |
0.390 |
0.576 |
0.523 |
0.476 |
0.538 |
0.532 |
SFE |
4.310 |
2.428 |
3.123 |
3.767 |
2.834 |
3.078 |
3.185 |
Sources: RBI, MOSPI and Authors’ calculations. |
Table A4 (II): Performances of Nowcasts: Values of Errors for Nowcasts vis-à-vis Realised Inflation Values (contd.) |
Data |
Measures of Forecast Error |
BR |
KL |
CH |
JH |
CHH |
AC |
IESH |
Bias |
1.580 |
0.915 |
5.555 |
1.598 |
3.151 |
3.569 |
MSE |
6.629 |
1.617 |
32.416 |
6.567 |
15.075 |
13.517 |
RMSE |
2.575 |
1.272 |
5.694 |
2.563 |
3.883 |
3.677 |
MAE |
2.421 |
1.127 |
6.729 |
2.363 |
3.701 |
4.006 |
MAPE |
0.362 |
0.222 |
1.209 |
0.420 |
1.099 |
0.627 |
SFE |
2.083 |
0.905 |
1.280 |
2.053 |
2.324 |
0.904 |
IESH-BA |
Bias |
-1.96 |
-3.04 |
1.08 |
-0.70 |
0.29 |
-0.73 |
MSE |
7.955 |
10.032 |
2.718 |
4.507 |
5.230 |
1.318 |
RMSE |
2.820 |
3.167 |
1.649 |
2.123 |
2.287 |
1.148 |
MAE |
2.523 |
3.351 |
1.432 |
1.909 |
1.959 |
1.012 |
MAPE |
0.289 |
0.535 |
0.323 |
0.292 |
0.636 |
0.160 |
SFE |
2.083 |
0.905 |
1.280 |
2.053 |
2.324 |
0.904 |
Reg-based |
Bias |
-1.521 |
-0.667 |
-0.631 |
-0.704 |
-0.400 |
-0.896 |
MSE |
6.817 |
1.249 |
1.643 |
4.017 |
3.324 |
1.620 |
RMSE |
2.611 |
1.118 |
1.282 |
2.004 |
1.823 |
1.273 |
MAE |
2.240 |
0.923 |
1.138 |
1.799 |
1.605 |
1.121 |
MAPE |
0.259 |
0.158 |
0.234 |
0.272 |
0.433 |
0.173 |
SFE |
2.175 |
0.919 |
1.143 |
1.923 |
1.823 |
0.927 |
1 IESH 12 CPI-U |
D |
Bias |
0.997 |
0.530 |
0.574 |
0.212 |
0.726 |
0.196 |
MSE |
5.522 |
1.271 |
1.441 |
3.396 |
3.369 |
1.050 |
RMSE |
2.350 |
1.128 |
1.200 |
1.843 |
1.836 |
1.025 |
MAE |
1.997 |
0.805 |
0.988 |
1.627 |
1.487 |
0.858 |
MAPE |
0.289 |
0.161 |
0.232 |
0.276 |
0.450 |
0.158 |
SFE |
2.180 |
1.020 |
1.080 |
1.876 |
1.728 |
1.031 |
D+C |
Bias |
4.598 |
4.197 |
2.714 |
4.830 |
3.547 |
3.035 |
MSE |
27.220 |
20.359 |
11.949 |
31.137 |
17.186 |
10.715 |
RMSE |
5.217 |
4.512 |
3.457 |
5.580 |
4.146 |
3.273 |
MAE |
5.609 |
4.914 |
3.191 |
6.309 |
4.494 |
3.379 |
MAPE |
0.727 |
0.782 |
0.591 |
0.935 |
1.053 |
0.544 |
SFE |
2.526 |
1.697 |
2.193 |
2.863 |
2.199 |
1.258 |
1 IESH 6 CPI-U |
D |
Bias |
0.559 |
0.198 |
0.299 |
-0.056 |
0.450 |
-0.031 |
MSE |
3.792 |
1.176 |
1.427 |
3.687 |
3.123 |
1.011 |
RMSE |
1.947 |
1.085 |
1.195 |
1.920 |
1.767 |
1.005 |
MAE |
1.725 |
0.802 |
0.938 |
1.673 |
1.390 |
0.846 |
MAPE |
0.251 |
0.164 |
0.216 |
0.269 |
0.376 |
0.151 |
SFE |
1.911 |
1.093 |
1.185 |
1.967 |
1.751 |
1.030 |
D+C |
Bias |
3.708 |
3.171 |
2.070 |
4.027 |
2.751 |
2.424 |
MSE |
25.016 |
14.265 |
9.681 |
26.874 |
13.844 |
8.046 |
RMSE |
5.002 |
3.777 |
3.111 |
5.184 |
3.721 |
2.836 |
MAE |
4.971 |
3.807 |
2.669 |
5.631 |
3.759 |
2.686 |
MAPE |
0.646 |
0.625 |
0.488 |
0.819 |
0.831 |
0.438 |
SFE |
3.440 |
2.102 |
2.380 |
3.345 |
2.567 |
1.509 |
All IESH All CPI-U |
D |
Bias |
-0.394 |
-0.679 |
0.000 |
0.712 |
-0.007 |
-1.041 |
MSE |
3.506 |
1.183 |
0.833 |
3.149 |
2.352 |
2.218 |
RMSE |
1.872 |
1.088 |
0.913 |
1.775 |
1.534 |
1.489 |
MAE |
1.429 |
0.954 |
0.668 |
1.599 |
1.176 |
1.310 |
MAPE |
0.189 |
0.170 |
0.164 |
0.285 |
0.302 |
0.199 |
SFE |
1.876 |
0.871 |
0.935 |
1.665 |
1.572 |
1.091 |
D+C |
Bias |
2.111 |
1.837 |
2.103 |
3.530 |
1.872 |
1.323 |
MSE |
11.957 |
7.306 |
7.757 |
19.020 |
8.438 |
3.604 |
RMSE |
3.458 |
2.703 |
2.785 |
4.361 |
2.905 |
1.898 |
MAE |
3.296 |
2.527 |
2.649 |
4.379 |
2.693 |
1.571 |
MAPE |
0.468 |
0.440 |
0.542 |
0.681 |
0.629 |
0.271 |
SFE |
2.806 |
2.032 |
1.872 |
2.625 |
2.276 |
1.396 |
All IESH 12 CPI-U |
D |
Bias |
0.879 |
-0.197 |
0.330 |
1.351 |
0.410 |
-0.401 |
MSE |
5.014 |
0.767 |
0.913 |
4.496 |
2.579 |
1.050 |
RMSE |
2.239 |
0.876 |
0.956 |
2.120 |
1.606 |
1.025 |
MAE |
1.953 |
0.748 |
0.748 |
1.906 |
1.296 |
0.898 |
MAPE |
0.275 |
0.144 |
0.184 |
0.344 |
0.397 |
0.151 |
SFE |
2.110 |
0.875 |
0.919 |
1.675 |
1.591 |
0.966 |
D+C |
Bias |
5.332 |
2.866 |
2.530 |
5.922 |
2.920 |
2.385 |
MSE |
34.776 |
11.847 |
11.260 |
39.619 |
13.461 |
6.960 |
RMSE |
5.897 |
3.442 |
3.356 |
6.294 |
3.669 |
2.638 |
MAE |
6.636 |
3.457 |
3.071 |
7.403 |
3.781 |
2.595 |
MAPE |
0.833 |
0.565 |
0.562 |
1.063 |
0.919 |
0.431 |
SFE |
2.582 |
1.953 |
2.259 |
2.185 |
2.277 |
1.155 |
All IESH 6 CPI-U |
D |
Bias |
0.462 |
-0.648 |
-0.013 |
0.967 |
0.065 |
-0.537 |
MSE |
3.481 |
2.300 |
0.767 |
3.587 |
2.502 |
1.192 |
RMSE |
1.866 |
1.517 |
0.876 |
1.894 |
1.582 |
1.092 |
MAE |
1.584 |
1.138 |
0.705 |
1.720 |
1.274 |
0.938 |
MAPE |
0.224 |
0.200 |
0.153 |
0.303 |
0.319 |
0.151 |
SFE |
1.852 |
1.405 |
0.897 |
1.669 |
1.620 |
0.974 |
D+C |
Bias |
3.899 |
1.329 |
1.561 |
4.810 |
2.044 |
1.795 |
MSE |
27.144 |
8.765 |
7.575 |
30.323 |
11.186 |
5.185 |
RMSE |
5.210 |
2.961 |
2.752 |
5.507 |
3.345 |
2.277 |
MAE |
5.343 |
2.694 |
2.294 |
6.089 |
3.167 |
2.015 |
MAPE |
0.680 |
0.441 |
0.408 |
0.880 |
0.684 |
0.338 |
SFE |
3.541 |
2.711 |
2.323 |
2.747 |
2.713 |
1.435 |
Sources: RBI, MOSPI and Authors’ calculations. |
Table A5: Performances of Three-months-ahead Forecasts: Values of Errors for Forecasts vis-à-vis Realised Inflation Values |
Data |
Measures of Forecast Error |
GJ |
KT |
MP |
OD |
TN |
DL |
AS |
IESH |
Bias |
5.083 |
4.837 |
3.133 |
4.410 |
5.271 |
6.930 |
4.408 |
MSE |
28.956 |
24.939 |
11.578 |
23.472 |
29.601 |
50.524 |
24.081 |
RMSE |
5.381 |
4.994 |
3.403 |
4.845 |
5.441 |
7.108 |
4.907 |
MAE |
6.133 |
5.718 |
3.512 |
5.258 |
6.337 |
8.833 |
5.285 |
MAPE |
0.970 |
0.873 |
0.529 |
0.906 |
0.963 |
2.268 |
1.062 |
SFE |
1.811 |
1.274 |
1.360 |
2.055 |
1.380 |
1.618 |
2.210 |
IESH-BA |
Bias |
-2.12 |
2.81 |
-1.47 |
-0.69 |
-0.24 |
2.40 |
-0.47 |
MSE |
7.604 |
9.466 |
3.913 |
4.504 |
1.872 |
8.269 |
4.870 |
RMSE |
2.758 |
3.077 |
1.978 |
2.122 |
1.368 |
2.876 |
2.207 |
MAE |
2.496 |
3.115 |
1.767 |
1.770 |
1.167 |
2.771 |
1.955 |
MAPE |
0.361 |
0.519 |
0.238 |
0.286 |
0.197 |
0.915 |
0.434 |
SFE |
1.811 |
1.274 |
1.360 |
2.055 |
1.380 |
1.618 |
2.210 |
Reg-based |
Bias |
-1.38 |
0.79 |
-1.35 |
-1.23 |
-0.43 |
0.57 |
0.37 |
MSE |
3.085 |
1.630 |
3.453 |
3.907 |
2.104 |
2.889 |
3.530 |
RMSE |
1.756 |
1.277 |
1.858 |
1.977 |
1.451 |
1.700 |
1.879 |
MAE |
1.501 |
1.042 |
1.673 |
1.624 |
1.206 |
1.576 |
1.550 |
MAPE |
0.222 |
0.196 |
0.227 |
0.233 |
0.191 |
0.539 |
0.336 |
SFE |
1.115 |
1.029 |
1.311 |
1.587 |
1.418 |
1.640 |
1.888 |
1 IESH 12 CPI-U |
D |
Bias |
-0.121 |
-0.432 |
0.188 |
0.023 |
0.201 |
-0.315 |
1.013 |
MSE |
2.801 |
2.114 |
2.713 |
3.852 |
2.101 |
4.063 |
7.283 |
RMSE |
1.674 |
1.454 |
1.647 |
1.963 |
1.449 |
2.016 |
2.699 |
MAE |
1.419 |
1.159 |
1.431 |
1.655 |
1.315 |
1.718 |
2.403 |
MAPE |
0.248 |
0.189 |
0.240 |
0.302 |
0.238 |
0.440 |
0.540 |
SFE |
1.711 |
1.423 |
1.677 |
2.011 |
1.471 |
2.040 |
2.563 |
D+C |
Bias |
1.745 |
1.363 |
3.245 |
2.285 |
2.889 |
1.354 |
3.545 |
MSE |
9.089 |
7.684 |
17.940 |
11.906 |
16.183 |
9.859 |
29.941 |
RMSE |
3.015 |
2.772 |
4.236 |
3.451 |
4.023 |
3.140 |
5.472 |
MAE |
2.582 |
2.686 |
4.263 |
3.305 |
3.982 |
2.890 |
5.281 |
MAPE |
0.437 |
0.434 |
0.618 |
0.611 |
0.641 |
0.801 |
0.988 |
SFE |
2.519 |
2.473 |
2.790 |
2.650 |
2.869 |
2.903 |
4.271 |
1 IESH 6 CPI-U |
D |
Bias |
-0.313 |
-0.816 |
-0.237 |
-0.334 |
0.018 |
-0.433 |
0.617 |
MSE |
2.785 |
2.825 |
3.438 |
4.828 |
2.331 |
3.658 |
6.746 |
RMSE |
1.669 |
1.681 |
1.854 |
2.197 |
1.527 |
1.913 |
2.597 |
MAE |
1.320 |
1.354 |
1.571 |
1.831 |
1.363 |
1.543 |
2.358 |
MAPE |
0.220 |
0.209 |
0.249 |
0.317 |
0.245 |
0.387 |
0.500 |
SFE |
1.680 |
1.506 |
1.884 |
2.225 |
1.564 |
1.909 |
2.585 |
D+C |
Bias |
1.384 |
0.433 |
2.065 |
0.970 |
1.395 |
0.684 |
3.110 |
MSE |
9.204 |
6.575 |
16.271 |
8.108 |
7.846 |
7.436 |
31.944 |
RMSE |
3.034 |
2.564 |
4.034 |
2.848 |
2.801 |
2.727 |
5.652 |
MAE |
2.673 |
2.423 |
3.926 |
2.539 |
2.290 |
2.269 |
5.571 |
MAPE |
0.450 |
0.398 |
0.581 |
0.459 |
0.398 |
0.587 |
0.959 |
SFE |
2.766 |
2.590 |
3.550 |
2.743 |
2.489 |
2.705 |
4.836 |
All IESH All CPI-U |
D |
Bias |
-1.532 |
-0.861 |
-1.476 |
-1.739 |
-1.403 |
-0.063 |
-1.909 |
MSE |
4.932 |
2.308 |
5.293 |
6.931 |
4.777 |
2.565 |
8.396 |
RMSE |
2.221 |
1.519 |
2.301 |
2.633 |
2.186 |
1.602 |
2.898 |
MAE |
2.145 |
1.212 |
2.118 |
2.489 |
1.948 |
1.375 |
2.520 |
MAPE |
0.345 |
0.186 |
0.296 |
0.380 |
0.280 |
0.415 |
0.383 |
SFE |
1.647 |
1.283 |
1.808 |
2.026 |
1.717 |
1.640 |
2.233 |
D+C |
Bias |
0.206 |
1.263 |
0.752 |
-0.030 |
0.742 |
1.695 |
-0.893 |
MSE |
4.693 |
5.360 |
9.871 |
6.532 |
6.008 |
7.802 |
7.165 |
RMSE |
2.166 |
2.315 |
3.142 |
2.556 |
2.451 |
2.793 |
2.677 |
MAE |
1.709 |
2.095 |
2.688 |
2.163 |
2.124 |
2.311 |
2.409 |
MAPE |
0.294 |
0.353 |
0.417 |
0.377 |
0.376 |
0.716 |
0.448 |
SFE |
2.210 |
1.988 |
3.126 |
2.619 |
2.394 |
2.275 |
2.586 |
All IESH 12 CPI-U |
D |
Bias |
-1.265 |
-0.546 |
-0.424 |
-0.794 |
-0.468 |
-0.419 |
-0.040 |
MSE |
3.529 |
2.072 |
2.373 |
3.870 |
2.337 |
4.002 |
6.412 |
RMSE |
1.879 |
1.439 |
1.540 |
1.967 |
1.529 |
2.001 |
2.532 |
MAE |
1.696 |
1.171 |
1.363 |
1.612 |
1.366 |
1.711 |
2.351 |
MAPE |
0.270 |
0.187 |
0.211 |
0.246 |
0.219 |
0.426 |
0.496 |
SFE |
1.424 |
1.365 |
1.517 |
1.844 |
1.491 |
2.004 |
2.594 |
D+C |
Bias |
0.545 |
1.705 |
2.585 |
1.418 |
2.498 |
1.374 |
1.828 |
MSE |
5.336 |
10.045 |
13.718 |
7.135 |
13.821 |
10.641 |
25.302 |
RMSE |
2.310 |
3.169 |
3.704 |
2.671 |
3.718 |
3.262 |
5.030 |
MAE |
1.969 |
3.113 |
3.653 |
2.441 |
3.687 |
2.981 |
4.865 |
MAPE |
0.363 |
0.492 |
0.535 |
0.448 |
0.592 |
0.812 |
0.889 |
SFE |
2.300 |
2.738 |
2.718 |
2.320 |
2.821 |
3.032 |
4.802 |
All IESH 6 CPI-U |
D |
Bias |
-1.251 |
-0.960 |
-0.521 |
-1.048 |
-0.796 |
-0.497 |
-0.435 |
MSE |
4.118 |
2.994 |
2.955 |
5.175 |
2.703 |
3.509 |
7.298 |
RMSE |
2.029 |
1.730 |
1.719 |
2.275 |
1.644 |
1.873 |
2.701 |
MAE |
1.845 |
1.409 |
1.398 |
1.933 |
1.470 |
1.551 |
2.460 |
MAPE |
0.294 |
0.216 |
0.210 |
0.299 |
0.233 |
0.388 |
0.468 |
SFE |
1.638 |
1.475 |
1.678 |
2.069 |
1.474 |
1.851 |
2.732 |
D+C |
Bias |
0.271 |
0.659 |
1.736 |
0.142 |
0.688 |
0.806 |
1.276 |
MSE |
6.707 |
8.404 |
12.766 |
6.516 |
6.400 |
7.635 |
26.119 |
RMSE |
2.590 |
2.899 |
3.573 |
2.553 |
2.530 |
2.763 |
5.111 |
MAE |
2.400 |
2.769 |
3.330 |
2.092 |
2.164 |
2.378 |
4.845 |
MAPE |
0.423 |
0.443 |
0.498 |
0.363 |
0.376 |
0.614 |
0.794 |
SFE |
2.639 |
2.893 |
3.200 |
2.612 |
2.495 |
2.708 |
5.071 |
Sources: RBI, MOSPI and Authors’ calculations. |
Table A5 (I): Performances of Three-months-ahead Forecasts: Values of Errors for Forecasts vis-à-vis Realised Inflation Values (contd.) |
Data |
Measures of Forecast Error |
TL |
RJ |
JK |
WB |
UP |
MHM |
MHN |
IESH |
Bias |
4.326 |
4.401 |
2.347 |
5.585 |
5.008 |
4.740 |
4.597 |
MSE |
20.988 |
23.603 |
29.136 |
34.387 |
26.435 |
24.188 |
22.076 |
RMSE |
4.581 |
4.858 |
5.398 |
5.864 |
5.142 |
4.918 |
4.699 |
MAE |
5.062 |
5.315 |
6.110 |
6.850 |
5.945 |
5.594 |
5.362 |
MAPE |
0.653 |
0.957 |
0.926 |
1.074 |
0.937 |
0.826 |
0.782 |
SFE |
1.545 |
2.109 |
4.981 |
1.830 |
1.193 |
1.343 |
0.994 |
IESH-BA |
Bias |
-0.24 |
-0.11 |
|
-0.28 |
0.29 |
-0.14 |
0.06 |
MSE |
2.328 |
4.248 |
|
3.267 |
1.438 |
1.736 |
0.944 |
RMSE |
1.526 |
2.061 |
|
1.807 |
1.199 |
1.318 |
0.971 |
MAE |
1.296 |
1.701 |
|
1.576 |
0.947 |
1.123 |
0.797 |
MAPE |
0.193 |
0.324 |
|
0.260 |
0.182 |
0.191 |
0.138 |
SFE |
1.545 |
2.109 |
|
1.830 |
1.193 |
1.343 |
0.994 |
Reg-based |
Bias |
-1.33 |
-0.68 |
|
-0.33 |
-0.46 |
-0.99 |
-1.10 |
MSE |
2.894 |
2.738 |
|
3.513 |
1.136 |
2.381 |
2.356 |
RMSE |
1.701 |
1.655 |
|
1.874 |
1.066 |
1.543 |
1.535 |
MAE |
1.500 |
1.412 |
|
1.600 |
0.922 |
1.360 |
1.328 |
MAPE |
0.190 |
0.244 |
|
0.256 |
0.158 |
0.200 |
0.189 |
SFE |
1.093 |
1.546 |
|
1.891 |
0.987 |
1.212 |
1.095 |
1 IESH 12 CPI-U |
D |
Bias |
-0.270 |
0.281 |
0.218 |
0.127 |
0.438 |
-0.711 |
-0.033 |
MSE |
3.484 |
3.923 |
4.534 |
4.660 |
2.924 |
3.821 |
3.609 |
RMSE |
1.867 |
1.981 |
2.129 |
2.159 |
1.710 |
1.955 |
1.900 |
MAE |
1.629 |
1.813 |
1.813 |
1.801 |
1.456 |
1.788 |
1.707 |
MAPE |
0.226 |
0.336 |
0.326 |
0.288 |
0.272 |
0.275 |
0.277 |
SFE |
1.893 |
2.009 |
2.170 |
2.208 |
1.694 |
1.866 |
1.946 |
D+C |
Bias |
3.080 |
2.203 |
3.829 |
3.039 |
2.701 |
1.911 |
2.714 |
MSE |
21.555 |
10.554 |
29.002 |
19.389 |
11.698 |
12.563 |
15.157 |
RMSE |
4.643 |
3.249 |
5.385 |
4.403 |
3.420 |
3.544 |
3.893 |
MAE |
4.699 |
3.049 |
5.957 |
4.329 |
3.279 |
3.132 |
3.612 |
MAPE |
0.589 |
0.525 |
0.924 |
0.669 |
0.559 |
0.487 |
0.562 |
SFE |
3.560 |
2.447 |
3.880 |
3.265 |
2.150 |
3.059 |
2.860 |
1 IESH 6 CPI-U |
D |
Bias |
-1.530 |
-0.051 |
-0.038 |
0.125 |
-0.037 |
-0.574 |
-0.051 |
MSE |
11.995 |
6.861 |
3.811 |
4.679 |
2.578 |
3.397 |
3.353 |
RMSE |
3.463 |
2.619 |
1.952 |
2.163 |
1.606 |
1.843 |
1.831 |
MAE |
2.677 |
2.093 |
1.583 |
1.914 |
1.356 |
1.521 |
1.551 |
MAPE |
0.295 |
0.336 |
0.275 |
0.305 |
0.246 |
0.224 |
0.245 |
SFE |
3.184 |
2.684 |
2.000 |
2.213 |
1.645 |
1.795 |
1.876 |
D+C |
Bias |
0.554 |
1.831 |
2.848 |
2.776 |
1.686 |
2.383 |
2.790 |
MSE |
22.998 |
15.988 |
21.423 |
21.617 |
10.231 |
16.317 |
17.870 |
RMSE |
4.796 |
3.998 |
4.629 |
4.649 |
3.199 |
4.039 |
4.227 |
MAE |
4.714 |
3.724 |
4.811 |
4.152 |
2.967 |
3.852 |
3.994 |
MAPE |
0.561 |
0.612 |
0.744 |
0.563 |
0.496 |
0.562 |
0.581 |
SFE |
4.881 |
3.643 |
3.738 |
3.821 |
2.785 |
3.343 |
3.254 |
All IESH All CPI-U |
D |
Bias |
-3.082 |
-0.630 |
-1.037 |
-2.159 |
-1.131 |
-1.363 |
-1.414 |
MSE |
17.318 |
3.372 |
5.114 |
9.272 |
2.678 |
4.761 |
4.971 |
RMSE |
4.161 |
1.836 |
2.261 |
3.045 |
1.636 |
2.182 |
2.230 |
MAE |
3.993 |
1.534 |
2.115 |
2.725 |
1.328 |
1.987 |
2.033 |
MAPE |
0.457 |
0.255 |
0.327 |
0.366 |
0.203 |
0.286 |
0.292 |
SFE |
2.865 |
1.767 |
2.059 |
2.200 |
1.212 |
1.746 |
1.766 |
D+C |
Bias |
-1.198 |
1.448 |
1.182 |
-0.763 |
0.645 |
1.728 |
1.614 |
MSE |
19.506 |
9.068 |
14.115 |
5.620 |
5.319 |
10.622 |
10.508 |
RMSE |
4.417 |
3.011 |
3.757 |
2.371 |
2.306 |
3.259 |
3.242 |
MAE |
3.924 |
2.832 |
3.393 |
1.982 |
1.999 |
2.691 |
2.695 |
MAPE |
0.439 |
0.530 |
0.556 |
0.285 |
0.331 |
0.397 |
0.399 |
SFE |
4.356 |
2.706 |
3.654 |
2.300 |
2.269 |
2.832 |
2.881 |
All IESH 12 CPI-U |
D |
Bias |
-1.063 |
-0.481 |
-0.113 |
-0.238 |
-0.461 |
-1.100 |
-1.077 |
MSE |
5.145 |
2.705 |
3.626 |
4.585 |
1.911 |
5.481 |
5.348 |
RMSE |
2.268 |
1.645 |
1.904 |
2.141 |
1.382 |
2.341 |
2.313 |
MAE |
1.858 |
1.459 |
1.597 |
1.746 |
1.230 |
1.939 |
1.896 |
MAPE |
0.240 |
0.254 |
0.274 |
0.269 |
0.216 |
0.276 |
0.268 |
SFE |
2.054 |
1.612 |
1.948 |
2.180 |
1.335 |
2.118 |
2.097 |
D+C |
Bias |
2.746 |
1.137 |
3.455 |
2.697 |
1.849 |
1.480 |
1.399 |
MSE |
22.461 |
4.075 |
25.775 |
17.086 |
7.834 |
12.501 |
11.763 |
RMSE |
4.739 |
2.019 |
5.077 |
4.134 |
2.799 |
3.536 |
3.430 |
MAE |
5.099 |
1.725 |
5.640 |
3.941 |
2.583 |
3.126 |
3.013 |
MAPE |
0.658 |
0.311 |
0.867 |
0.618 |
0.455 |
0.485 |
0.468 |
SFE |
3.958 |
1.709 |
3.812 |
3.210 |
2.153 |
3.291 |
3.209 |
All IESH 6 CPI-U |
D |
Bias |
-2.045 |
-0.833 |
-0.373 |
-0.325 |
-0.682 |
-0.730 |
-0.823 |
MSE |
13.132 |
3.827 |
3.405 |
4.958 |
2.820 |
3.451 |
3.745 |
RMSE |
3.624 |
1.956 |
1.845 |
2.227 |
1.679 |
1.858 |
1.935 |
MAE |
2.873 |
1.614 |
1.528 |
1.989 |
1.402 |
1.527 |
1.597 |
MAPE |
0.309 |
0.257 |
0.250 |
0.310 |
0.236 |
0.220 |
0.229 |
SFE |
3.066 |
1.814 |
1.852 |
2.257 |
1.573 |
1.750 |
1.795 |
D+C |
Bias |
0.357 |
0.796 |
2.484 |
2.414 |
0.994 |
1.990 |
1.859 |
MSE |
23.423 |
9.539 |
19.853 |
20.304 |
8.649 |
13.916 |
13.937 |
RMSE |
4.840 |
3.089 |
4.456 |
4.506 |
2.941 |
3.730 |
3.733 |
MAE |
4.774 |
2.561 |
4.614 |
3.979 |
2.655 |
3.429 |
3.460 |
MAPE |
0.572 |
0.454 |
0.704 |
0.545 |
0.445 |
0.504 |
0.511 |
SFE |
4.946 |
3.058 |
3.791 |
3.899 |
2.836 |
3.233 |
3.317 |
Sources: RBI, MOSPI and Authors’ calculations. |
Table A5 (II): Performances of Three-months-ahead Forecasts: Values of Errors for Forecasts vis-à-vis Realised Inflation Values (contd.) |
Data |
Measures of Forecast Error |
BR |
KL |
CH |
JH |
CHH |
AC |
IESH |
Bias |
2.415 |
2.533 |
5.807 |
2.362 |
4.095 |
4.763 |
MSE |
9.886 |
7.326 |
35.845 |
8.658 |
20.594 |
23.424 |
RMSE |
3.144 |
2.707 |
5.987 |
2.942 |
4.538 |
4.840 |
MAE |
3.065 |
2.749 |
7.121 |
2.694 |
4.831 |
5.577 |
MAPE |
0.457 |
0.491 |
1.391 |
0.489 |
1.272 |
0.840 |
SFE |
2.063 |
0.978 |
1.494 |
1.798 |
2.004 |
0.881 |
IESH-BA |
Bias |
-1.72 |
-1.53 |
1.35 |
-0.29 |
1.03 |
0.01 |
MSE |
7.019 |
3.249 |
3.950 |
3.162 |
4.879 |
0.740 |
RMSE |
2.649 |
1.803 |
1.987 |
1.778 |
2.209 |
0.860 |
MAE |
2.350 |
1.616 |
1.767 |
1.536 |
1.850 |
0.780 |
MAPE |
0.285 |
0.263 |
0.443 |
0.274 |
0.603 |
0.142 |
SFE |
2.063 |
0.978 |
1.494 |
1.798 |
2.004 |
0.881 |
Reg-based |
Bias |
-1.56 |
-0.28 |
-0.82 |
-0.56 |
-0.22 |
-0.63 |
MSE |
5.973 |
0.623 |
2.519 |
2.640 |
3.281 |
1.029 |
RMSE |
2.444 |
0.789 |
1.587 |
1.625 |
1.811 |
1.015 |
MAE |
1.975 |
0.665 |
1.384 |
1.385 |
1.582 |
0.859 |
MAPE |
0.223 |
0.124 |
0.286 |
0.231 |
0.457 |
0.136 |
SFE |
1.931 |
0.758 |
1.395 |
1.561 |
1.842 |
0.816 |
1 IESH 12 CPI-U |
D |
Bias |
1.134 |
0.504 |
0.619 |
0.914 |
0.764 |
0.024 |
MSE |
5.409 |
1.262 |
1.837 |
2.708 |
4.291 |
1.002 |
RMSE |
2.326 |
1.123 |
1.355 |
1.646 |
2.072 |
1.001 |
MAE |
1.989 |
0.897 |
1.070 |
1.454 |
1.770 |
0.861 |
MAPE |
0.293 |
0.179 |
0.292 |
0.264 |
0.559 |
0.154 |
SFE |
2.081 |
1.029 |
1.236 |
1.402 |
1.973 |
1.025 |
D+C |
Bias |
4.616 |
3.735 |
2.399 |
4.895 |
3.284 |
2.543 |
MSE |
28.566 |
18.297 |
9.581 |
30.804 |
18.343 |
7.786 |
RMSE |
5.345 |
4.278 |
3.095 |
5.550 |
4.283 |
2.790 |
MAE |
5.887 |
4.513 |
2.918 |
6.168 |
4.428 |
2.782 |
MAPE |
0.787 |
0.743 |
0.587 |
0.908 |
1.145 |
0.462 |
SFE |
2.760 |
2.136 |
2.005 |
2.681 |
2.817 |
1.176 |
1 IESH 6 CPI-U |
D |
Bias |
0.795 |
0.306 |
0.487 |
0.548 |
0.552 |
-0.219 |
MSE |
4.413 |
1.072 |
2.100 |
2.581 |
4.275 |
1.304 |
RMSE |
2.101 |
1.035 |
1.449 |
1.607 |
2.068 |
1.142 |
MAE |
1.847 |
0.877 |
1.213 |
1.481 |
1.733 |
0.969 |
MAPE |
0.271 |
0.176 |
0.305 |
0.259 |
0.535 |
0.164 |
SFE |
1.993 |
1.013 |
1.398 |
1.547 |
2.042 |
1.148 |
D+C |
Bias |
3.697 |
2.709 |
1.775 |
4.214 |
2.587 |
1.860 |
MSE |
25.930 |
12.144 |
8.470 |
26.945 |
14.302 |
5.865 |
RMSE |
5.092 |
3.485 |
2.910 |
5.191 |
3.782 |
2.422 |
MAE |
4.990 |
3.309 |
2.621 |
5.503 |
3.666 |
2.192 |
MAPE |
0.673 |
0.556 |
0.544 |
0.817 |
0.906 |
0.368 |
SFE |
3.588 |
2.246 |
2.363 |
3.106 |
2.826 |
1.589 |
All IESH All CPI-U |
D |
Bias |
-0.747 |
-0.533 |
-0.091 |
0.619 |
-0.257 |
-1.270 |
MSE |
3.785 |
1.224 |
1.443 |
2.283 |
2.963 |
2.870 |
RMSE |
1.945 |
1.106 |
1.201 |
1.511 |
1.721 |
1.694 |
MAE |
1.578 |
0.985 |
0.944 |
1.303 |
1.413 |
1.525 |
MAPE |
0.202 |
0.178 |
0.245 |
0.243 |
0.396 |
0.236 |
SFE |
1.841 |
0.994 |
1.227 |
1.412 |
1.744 |
1.148 |
D+C |
Bias |
1.490 |
1.925 |
1.769 |
3.277 |
1.414 |
0.898 |
MSE |
10.018 |
9.310 |
7.080 |
17.796 |
7.333 |
2.720 |
RMSE |
3.165 |
3.051 |
2.661 |
4.219 |
2.708 |
1.649 |
MAE |
2.902 |
2.940 |
2.581 |
4.017 |
2.530 |
1.323 |
MAPE |
0.421 |
0.524 |
0.604 |
0.652 |
0.676 |
0.230 |
SFE |
2.862 |
2.426 |
2.036 |
2.722 |
2.366 |
1.418 |
All IESH 12 CPI-U |
D |
Bias |
0.714 |
-0.081 |
0.346 |
1.313 |
0.164 |
-0.598 |
MSE |
5.004 |
0.972 |
1.024 |
3.231 |
3.300 |
1.301 |
RMSE |
2.237 |
0.986 |
1.012 |
1.797 |
1.817 |
1.141 |
MAE |
1.782 |
0.814 |
0.766 |
1.634 |
1.492 |
0.979 |
MAPE |
0.254 |
0.160 |
0.221 |
0.299 |
0.455 |
0.157 |
SFE |
2.173 |
1.007 |
0.974 |
1.258 |
1.854 |
0.995 |
D+C |
Bias |
4.598 |
2.773 |
2.256 |
5.603 |
2.358 |
1.956 |
MSE |
28.085 |
12.929 |
8.602 |
34.612 |
13.339 |
5.088 |
RMSE |
5.300 |
3.596 |
2.933 |
5.883 |
3.652 |
2.256 |
MAE |
5.885 |
3.674 |
2.671 |
6.877 |
3.654 |
2.144 |
MAPE |
0.763 |
0.622 |
0.542 |
1.006 |
0.981 |
0.366 |
SFE |
2.700 |
2.346 |
1.921 |
1.838 |
2.858 |
1.152 |
All IESH 6 CPI-U |
D |
Bias |
0.413 |
-0.520 |
-0.088 |
1.047 |
-0.110 |
-0.744 |
MSE |
3.853 |
2.580 |
1.505 |
2.686 |
2.898 |
1.676 |
RMSE |
1.963 |
1.606 |
1.227 |
1.639 |
1.702 |
1.294 |
MAE |
1.651 |
1.194 |
0.997 |
1.528 |
1.381 |
1.039 |
MAPE |
0.237 |
0.209 |
0.258 |
0.277 |
0.396 |
0.160 |
SFE |
1.966 |
1.557 |
1.254 |
1.292 |
1.741 |
1.085 |
D+C |
Bias |
3.171 |
1.344 |
1.237 |
4.644 |
1.625 |
1.296 |
MSE |
23.391 |
10.131 |
6.982 |
27.945 |
10.204 |
3.747 |
RMSE |
4.836 |
3.183 |
2.642 |
5.286 |
3.194 |
1.936 |
MAE |
4.596 |
2.933 |
2.387 |
5.687 |
3.033 |
1.666 |
MAPE |
0.627 |
0.497 |
0.502 |
0.847 |
0.745 |
0.286 |
SFE |
3.742 |
2.956 |
2.393 |
2.587 |
2.818 |
1.473 |
Sources: RBI, MOSPI and Authors’ calculations. |
Table A6: Performances of One-year-ahead Forecasts: Values of Errors for Forecasts vis-à-vis Realised Inflation Values |
Data |
Measures of Forecast Error |
GJ |
KT |
MP |
OD |
TN |
DL |
AS |
IESH |
Bias |
5.163 |
4.690 |
2.972 |
3.872 |
5.437 |
6.010 |
4.135 |
MSE |
28.458 |
24.453 |
10.652 |
18.172 |
31.928 |
42.197 |
25.075 |
RMSE |
5.335 |
4.945 |
3.264 |
4.263 |
5.650 |
6.496 |
5.008 |
MAE |
6.183 |
5.560 |
3.318 |
4.511 |
6.598 |
7.604 |
5.306 |
MAPE |
1.000 |
0.864 |
0.535 |
0.815 |
1.007 |
2.065 |
1.100 |
SFE |
1.377 |
1.607 |
1.383 |
1.827 |
1.578 |
2.527 |
2.895 |
IESH-BA |
Bias |
-3.45 |
1.63 |
-2.57 |
-3.10 |
-1.64 |
1.18 |
-3.71 |
MSE |
13.700 |
5.118 |
8.407 |
12.804 |
5.073 |
7.476 |
21.722 |
RMSE |
3.701 |
2.262 |
2.900 |
3.578 |
2.252 |
2.734 |
4.661 |
MAE |
3.906 |
1.969 |
2.835 |
3.545 |
2.082 |
2.509 |
4.690 |
MAPE |
0.603 |
0.356 |
0.381 |
0.510 |
0.299 |
0.827 |
0.707 |
SFE |
1.377 |
1.607 |
1.383 |
1.827 |
1.578 |
2.527 |
2.895 |
Reg-based |
Bias |
-1.10 |
-0.01 |
-1.17 |
-0.77 |
-0.62 |
0.09 |
0.95 |
MSE |
2.535 |
1.287 |
3.245 |
3.640 |
2.332 |
2.928 |
2.620 |
RMSE |
1.592 |
1.134 |
1.801 |
1.908 |
1.527 |
1.711 |
1.619 |
MAE |
1.414 |
0.922 |
1.579 |
1.556 |
1.255 |
1.531 |
1.477 |
MAPE |
0.230 |
0.174 |
0.224 |
0.244 |
0.191 |
0.481 |
0.335 |
SFE |
1.179 |
1.162 |
1.399 |
1.790 |
1.430 |
1.751 |
1.346 |
1 IESH 12 CPI-U |
D |
Bias |
-0.600 |
-0.662 |
0.089 |
0.102 |
-0.171 |
-0.412 |
1.971 |
MSE |
3.102 |
2.617 |
2.981 |
2.608 |
3.354 |
5.574 |
13.016 |
RMSE |
1.761 |
1.618 |
1.726 |
1.615 |
1.831 |
2.361 |
3.608 |
MAE |
1.536 |
1.420 |
1.565 |
1.363 |
1.583 |
2.224 |
3.417 |
MAPE |
0.265 |
0.238 |
0.260 |
0.258 |
0.256 |
0.602 |
0.744 |
SFE |
1.697 |
1.512 |
1.767 |
1.651 |
1.868 |
2.382 |
3.097 |
D+C |
Bias |
0.976 |
1.216 |
2.817 |
2.501 |
2.531 |
1.217 |
4.623 |
MSE |
7.244 |
7.699 |
16.138 |
12.415 |
15.662 |
11.575 |
46.827 |
RMSE |
2.691 |
2.775 |
4.017 |
3.524 |
3.958 |
3.402 |
6.843 |
MAE |
2.489 |
2.528 |
3.852 |
3.233 |
3.795 |
3.095 |
7.070 |
MAPE |
0.436 |
0.441 |
0.596 |
0.618 |
0.616 |
0.956 |
1.331 |
SFE |
2.570 |
2.556 |
2.934 |
2.543 |
3.117 |
3.255 |
5.170 |
1 IESH 6 CPI-U |
D |
Bias |
-0.456 |
-0.706 |
-0.217 |
0.000 |
-0.319 |
-0.424 |
1.614 |
MSE |
2.800 |
2.432 |
3.873 |
1.805 |
2.665 |
6.551 |
13.555 |
RMSE |
1.673 |
1.560 |
1.968 |
1.344 |
1.633 |
2.559 |
3.682 |
MAE |
1.452 |
1.328 |
1.784 |
1.106 |
1.367 |
2.396 |
3.333 |
MAPE |
0.252 |
0.221 |
0.282 |
0.206 |
0.216 |
0.645 |
0.725 |
SFE |
1.650 |
1.425 |
2.004 |
1.377 |
1.641 |
2.586 |
3.391 |
D+C |
Bias |
0.936 |
0.663 |
2.057 |
1.606 |
0.794 |
0.827 |
4.627 |
MSE |
8.875 |
4.655 |
12.904 |
6.043 |
4.042 |
11.175 |
53.581 |
RMSE |
2.979 |
2.158 |
3.592 |
2.458 |
2.010 |
3.343 |
7.320 |
MAE |
2.618 |
1.913 |
3.483 |
2.264 |
1.661 |
3.100 |
7.642 |
MAPE |
0.448 |
0.335 |
0.541 |
0.426 |
0.275 |
0.869 |
1.439 |
SFE |
2.898 |
2.104 |
3.018 |
1.907 |
1.893 |
3.319 |
5.812 |
All IESH All CPI-U |
D |
Bias |
-1.880 |
-0.775 |
-1.616 |
-1.758 |
-1.891 |
0.047 |
-1.785 |
MSE |
5.699 |
2.071 |
5.691 |
5.137 |
5.232 |
3.740 |
5.956 |
RMSE |
2.387 |
1.439 |
2.385 |
2.266 |
2.287 |
1.934 |
2.440 |
MAE |
2.267 |
1.304 |
2.377 |
2.010 |
2.066 |
1.766 |
2.117 |
MAPE |
0.359 |
0.219 |
0.348 |
0.294 |
0.283 |
0.528 |
0.359 |
SFE |
1.507 |
1.242 |
1.798 |
1.466 |
1.319 |
1.981 |
1.705 |
D+C |
Bias |
-0.384 |
1.177 |
0.190 |
-0.071 |
-0.223 |
2.123 |
-0.404 |
MSE |
2.919 |
3.330 |
5.318 |
3.768 |
1.312 |
9.312 |
4.142 |
RMSE |
1.708 |
1.825 |
2.306 |
1.941 |
1.145 |
3.052 |
2.035 |
MAE |
1.428 |
1.570 |
1.841 |
1.608 |
0.932 |
2.668 |
1.653 |
MAPE |
0.240 |
0.278 |
0.301 |
0.292 |
0.156 |
0.824 |
0.355 |
SFE |
1.706 |
1.429 |
2.355 |
1.988 |
1.151 |
2.246 |
2.044 |
All IESH 12 CPI-U |
D |
Bias |
-1.570 |
-0.545 |
-0.562 |
-0.652 |
-0.796 |
-0.621 |
0.575 |
MSE |
4.780 |
2.231 |
3.606 |
3.722 |
4.355 |
6.188 |
9.745 |
RMSE |
2.186 |
1.494 |
1.899 |
1.929 |
2.087 |
2.488 |
3.122 |
MAE |
1.788 |
1.316 |
1.618 |
1.540 |
1.809 |
2.352 |
2.975 |
MAPE |
0.259 |
0.228 |
0.241 |
0.243 |
0.269 |
0.617 |
0.671 |
SFE |
1.559 |
1.425 |
1.858 |
1.861 |
1.977 |
2.468 |
3.144 |
D+C |
Bias |
-0.162 |
1.377 |
1.796 |
1.132 |
1.789 |
0.989 |
2.938 |
MSE |
6.111 |
10.498 |
13.298 |
8.509 |
14.051 |
12.704 |
35.379 |
RMSE |
2.472 |
3.240 |
3.647 |
2.917 |
3.748 |
3.564 |
5.948 |
|
|
MAE |
2.170 |
3.083 |
3.466 |
2.618 |
3.592 |
3.252 |
5.861 |
MAPE |
0.369 |
0.525 |
0.533 |
0.511 |
0.590 |
0.973 |
1.143 |
SFE |
2.528 |
3.005 |
3.252 |
2.755 |
3.375 |
3.509 |
5.299 |
All IESH 6 CPI-U |
D |
Bias |
-1.343 |
-0.829 |
-0.627 |
-0.831 |
-1.254 |
-0.722 |
0.390 |
MSE |
4.177 |
2.843 |
4.107 |
3.776 |
4.296 |
6.883 |
11.655 |
RMSE |
2.044 |
1.686 |
2.027 |
1.943 |
2.073 |
2.624 |
3.414 |
MAE |
1.779 |
1.480 |
1.895 |
1.587 |
1.782 |
2.477 |
3.190 |
MAPE |
0.282 |
0.245 |
0.288 |
0.250 |
0.253 |
0.620 |
0.714 |
SFE |
1.579 |
1.505 |
1.975 |
1.800 |
1.691 |
2.585 |
3.475 |
D+C |
Bias |
-0.064 |
0.345 |
0.872 |
0.061 |
-0.639 |
0.493 |
2.632 |
MSE |
7.555 |
6.253 |
8.794 |
4.836 |
3.511 |
11.625 |
41.758 |
RMSE |
2.749 |
2.501 |
2.966 |
2.199 |
1.874 |
3.410 |
6.462 |
MAE |
2.523 |
2.259 |
2.782 |
1.953 |
1.528 |
3.184 |
6.326 |
MAPE |
0.436 |
0.370 |
0.435 |
0.350 |
0.240 |
0.841 |
1.259 |
SFE |
2.816 |
2.538 |
2.904 |
2.253 |
1.805 |
3.457 |
6.048 |
Sources: RBI, MOSPI and Authors’ calculations. |
Table A6 (I): Performances of One-year-ahead Forecasts: Values of Errors for Forecasts vis-à-vis Realised Inflation Values (contd.) |
Data |
Measures of Forecast Error |
TL |
RJ |
JK |
WB |
UP |
MHM |
MHN |
IESH |
Bias |
4.326 |
3.749 |
-0.048 |
5.353 |
4.491 |
3.526 |
4.224 |
MSE |
20.271 |
17.831 |
34.492 |
32.305 |
21.448 |
14.892 |
21.148 |
RMSE |
4.502 |
4.223 |
5.873 |
5.684 |
4.631 |
3.859 |
4.599 |
MAE |
5.027 |
4.393 |
6.649 |
6.540 |
5.235 |
4.035 |
5.034 |
MAPE |
0.657 |
0.843 |
1.013 |
1.094 |
0.841 |
0.634 |
0.775 |
SFE |
1.279 |
1.992 |
6.018 |
1.956 |
1.158 |
1.607 |
1.864 |
IESH-BA |
Bias |
-1.67 |
-2.65 |
|
-2.07 |
-1.74 |
-2.70 |
-1.95 |
MSE |
4.335 |
10.812 |
|
7.945 |
4.304 |
9.730 |
7.113 |
RMSE |
2.082 |
3.288 |
|
2.819 |
2.075 |
3.119 |
2.667 |
MAE |
1.846 |
3.097 |
|
2.588 |
1.874 |
3.021 |
2.349 |
MAPE |
0.237 |
0.462 |
|
0.358 |
0.297 |
0.423 |
0.319 |
SFE |
1.279 |
1.992 |
|
1.956 |
1.158 |
1.607 |
1.864 |
Reg-based |
Bias |
-1.19 |
-0.86 |
|
-0.33 |
-0.84 |
-1.38 |
-1.39 |
MSE |
2.470 |
3.532 |
|
2.030 |
1.910 |
3.344 |
3.234 |
RMSE |
1.572 |
1.879 |
|
1.425 |
1.382 |
1.829 |
1.798 |
MAE |
1.372 |
1.573 |
|
1.120 |
1.165 |
1.577 |
1.555 |
MAPE |
0.179 |
0.268 |
|
0.194 |
0.193 |
0.222 |
0.218 |
SFE |
1.049 |
1.713 |
|
1.419 |
1.124 |
1.234 |
1.167 |
1 IESH 12 CPI-U |
D |
Bias |
-0.726 |
-0.316 |
0.490 |
-0.089 |
0.227 |
-1.050 |
-0.301 |
MSE |
2.234 |
2.909 |
1.913 |
6.020 |
2.997 |
3.595 |
2.337 |
RMSE |
1.495 |
1.706 |
1.383 |
2.454 |
1.731 |
1.896 |
1.529 |
MAE |
1.297 |
1.341 |
1.027 |
2.008 |
1.562 |
1.713 |
1.353 |
MAPE |
0.174 |
0.263 |
0.215 |
0.342 |
0.283 |
0.255 |
0.222 |
SFE |
1.815 |
1.717 |
1.326 |
2.513 |
1.758 |
1.996 |
2.025 |
D+C |
Bias |
1.803 |
1.737 |
4.832 |
2.444 |
2.500 |
0.907 |
1.291 |
MSE |
10.991 |
7.107 |
31.625 |
17.590 |
10.407 |
9.150 |
9.878 |
RMSE |
3.315 |
2.666 |
5.624 |
4.194 |
3.226 |
3.025 |
3.143 |
MAE |
3.159 |
2.462 |
6.136 |
4.252 |
3.111 |
2.588 |
2.725 |
MAPE |
0.424 |
0.506 |
0.895 |
0.730 |
0.534 |
0.415 |
0.428 |
SFE |
2.851 |
2.072 |
2.948 |
3.493 |
2.089 |
2.957 |
2.936 |
1 IESH 6 CPI-U |
D |
Bias |
-1.602 |
-0.203 |
0.426 |
0.296 |
-0.064 |
-0.866 |
-0.008 |
MSE |
9.231 |
3.095 |
2.632 |
7.098 |
4.179 |
4.670 |
3.395 |
RMSE |
3.038 |
1.759 |
1.622 |
2.664 |
2.044 |
2.161 |
1.843 |
MAE |
2.453 |
1.442 |
1.130 |
2.238 |
1.875 |
1.925 |
1.653 |
MAPE |
0.287 |
0.280 |
0.242 |
0.383 |
0.330 |
0.290 |
0.279 |
SFE |
2.645 |
1.791 |
1.604 |
2.713 |
2.094 |
2.029 |
1.888 |
D+C |
Bias |
0.643 |
1.921 |
4.107 |
3.023 |
1.909 |
2.044 |
2.317 |
MSE |
15.796 |
9.976 |
27.630 |
27.811 |
13.785 |
14.274 |
16.841 |
RMSE |
3.974 |
3.158 |
5.256 |
5.274 |
3.713 |
3.778 |
4.104 |
MAE |
3.640 |
2.918 |
5.313 |
5.052 |
3.639 |
3.509 |
3.893 |
MAPE |
0.466 |
0.557 |
0.843 |
0.755 |
0.608 |
0.535 |
0.579 |
SFE |
4.019 |
2.569 |
3.362 |
4.428 |
3.263 |
3.256 |
3.471 |
All IESH All CPI-U |
D |
Bias |
-3.574 |
-0.918 |
-0.881 |
-2.126 |
-1.508 |
-1.641 |
-1.700 |
MSE |
17.987 |
3.459 |
5.403 |
10.068 |
4.368 |
6.692 |
6.934 |
RMSE |
4.241 |
1.860 |
2.325 |
3.173 |
2.090 |
2.587 |
2.633 |
MAE |
4.368 |
1.575 |
2.137 |
3.033 |
1.913 |
2.505 |
2.569 |
MAPE |
0.525 |
0.269 |
0.375 |
0.437 |
0.295 |
0.365 |
0.375 |
SFE |
2.339 |
1.657 |
2.204 |
2.414 |
1.483 |
2.049 |
2.061 |
D+C |
Bias |
-2.097 |
1.360 |
1.541 |
-0.814 |
0.403 |
1.245 |
0.893 |
MSE |
14.314 |
6.306 |
15.392 |
6.712 |
6.162 |
10.759 |
9.953 |
RMSE |
3.783 |
2.511 |
3.923 |
2.591 |
2.482 |
3.280 |
3.155 |
MAE |
3.402 |
2.302 |
3.080 |
2.413 |
1.909 |
2.586 |
2.480 |
MAPE |
0.404 |
0.480 |
0.579 |
0.383 |
0.325 |
0.396 |
0.378 |
SFE |
3.227 |
2.163 |
3.697 |
2.520 |
2.510 |
3.110 |
3.101 |
All IESH 12 CPI-U |
D |
Bias |
-1.633 |
-1.136 |
0.316 |
-0.480 |
-0.810 |
-1.449 |
-1.547 |
MSE |
5.597 |
4.159 |
1.864 |
6.317 |
2.536 |
5.469 |
5.840 |
RMSE |
2.366 |
2.039 |
1.365 |
2.513 |
1.593 |
2.339 |
2.417 |
MAE |
2.002 |
1.750 |
1.069 |
2.060 |
1.315 |
2.039 |
2.124 |
MAPE |
0.254 |
0.296 |
0.227 |
0.340 |
0.209 |
0.292 |
0.304 |
SFE |
1.754 |
1.735 |
1.361 |
2.528 |
1.405 |
1.881 |
1.902 |
D+C |
Bias |
0.967 |
0.331 |
4.402 |
1.976 |
1.348 |
0.590 |
0.021 |
MSE |
10.472 |
4.410 |
27.701 |
15.957 |
5.959 |
9.557 |
9.071 |
RMSE |
3.236 |
2.100 |
5.263 |
3.995 |
2.441 |
3.091 |
3.012 |
MAE |
3.030 |
1.920 |
5.781 |
3.933 |
2.173 |
2.725 |
2.658 |
MAPE |
0.410 |
0.401 |
0.865 |
0.683 |
0.384 |
0.431 |
0.415 |
SFE |
3.164 |
2.125 |
2.956 |
3.557 |
2.086 |
3.109 |
3.086 |
All IESH 6 CPI-U |
D |
Bias |
-2.417 |
-1.095 |
0.156 |
-0.068 |
-0.838 |
-0.909 |
-1.014 |
MSE |
12.984 |
4.783 |
3.140 |
7.400 |
3.974 |
4.226 |
4.509 |
RMSE |
3.603 |
2.187 |
1.772 |
2.720 |
1.993 |
2.056 |
2.123 |
MAE |
3.132 |
1.892 |
1.328 |
2.221 |
1.667 |
1.902 |
1.972 |
MAPE |
0.365 |
0.305 |
0.268 |
0.366 |
0.261 |
0.294 |
0.303 |
SFE |
2.738 |
1.940 |
1.809 |
2.787 |
1.853 |
1.889 |
1.912 |
D+C |
Bias |
-0.491 |
0.605 |
3.479 |
2.505 |
0.906 |
1.476 |
1.063 |
MSE |
18.092 |
7.700 |
25.397 |
24.707 |
10.289 |
13.695 |
13.591 |
RMSE |
4.253 |
2.775 |
5.040 |
4.971 |
3.208 |
3.701 |
3.687 |
MAE |
4.090 |
2.544 |
5.207 |
4.525 |
3.026 |
3.438 |
3.404 |
MAPE |
0.523 |
0.449 |
0.839 |
0.672 |
0.501 |
0.522 |
0.510 |
SFE |
4.329 |
2.775 |
3.736 |
4.399 |
3.153 |
3.478 |
3.617 |
Sources: RBI, MOSPI and Authors’ calculations. |
Table A6 (II): Performances of One-year-ahead Forecasts: Values of Errors for Forecasts vis-à-vis Realised Inflation Values (contd.) |
Data |
Measures of Forecast Error |
BR |
KL |
CH |
JH |
CHH |
AC |
IESH |
Bias |
2.165 |
3.730 |
4.817 |
1.687 |
4.212 |
4.429 |
MSE |
10.487 |
15.004 |
25.860 |
7.051 |
20.965 |
20.875 |
RMSE |
3.238 |
3.873 |
5.085 |
2.655 |
4.579 |
4.569 |
MAE |
3.125 |
4.226 |
5.743 |
2.412 |
4.956 |
5.150 |
MAPE |
0.464 |
0.738 |
1.098 |
0.454 |
1.238 |
0.806 |
SFE |
2.468 |
1.072 |
1.671 |
2.101 |
1.840 |
1.148 |
IESH-BA |
Bias |
-4.15 |
-2.88 |
-0.70 |
-2.04 |
0.91 |
-1.80 |
MSE |
23.041 |
9.382 |
3.157 |
8.350 |
4.060 |
4.511 |
RMSE |
4.800 |
3.063 |
1.777 |
2.890 |
2.015 |
2.124 |
MAE |
5.114 |
3.170 |
1.628 |
2.626 |
1.778 |
1.988 |
MAPE |
0.608 |
0.521 |
0.331 |
0.352 |
0.512 |
0.304 |
SFE |
2.468 |
1.072 |
1.671 |
2.101 |
1.840 |
1.149 |
Reg-based |
Bias |
-1.51 |
-0.02 |
-0.35 |
-0.18 |
0.72 |
-0.72 |
MSE |
5.498 |
0.614 |
1.305 |
2.004 |
2.796 |
1.260 |
RMSE |
2.345 |
0.784 |
1.142 |
1.416 |
1.672 |
1.123 |
MAE |
1.932 |
0.622 |
0.924 |
1.258 |
1.383 |
0.962 |
MAPE |
0.232 |
0.129 |
0.201 |
0.220 |
0.457 |
0.155 |
SFE |
1.837 |
0.803 |
1.115 |
1.439 |
1.546 |
0.879 |
1 IESH 12 CPI-U |
D |
Bias |
0.737 |
0.245 |
0.681 |
0.990 |
0.825 |
-0.329 |
MSE |
8.477 |
1.185 |
2.807 |
4.461 |
5.117 |
1.228 |
RMSE |
2.912 |
1.089 |
1.675 |
2.112 |
2.262 |
1.108 |
MAE |
2.489 |
0.932 |
1.409 |
1.978 |
2.025 |
0.947 |
MAPE |
0.332 |
0.192 |
0.336 |
0.360 |
0.640 |
0.166 |
SFE |
2.886 |
1.087 |
1.568 |
1.912 |
2.158 |
1.084 |
D+C |
Bias |
3.313 |
3.237 |
2.516 |
4.734 |
3.235 |
1.952 |
MSE |
23.211 |
14.532 |
15.213 |
31.946 |
18.216 |
5.836 |
RMSE |
4.818 |
3.812 |
3.900 |
5.652 |
4.268 |
2.416 |
MAE |
5.011 |
3.962 |
3.612 |
6.213 |
4.251 |
2.284 |
MAPE |
0.686 |
0.693 |
0.741 |
0.987 |
1.183 |
0.399 |
SFE |
3.584 |
2.063 |
3.054 |
3.165 |
2.852 |
1.458 |
1 IESH 6 CPI-U |
D |
Bias |
0.531 |
0.250 |
0.596 |
0.679 |
0.894 |
-0.266 |
MSE |
5.773 |
0.998 |
2.464 |
4.910 |
3.969 |
1.426 |
RMSE |
2.403 |
0.999 |
1.570 |
2.216 |
1.992 |
1.194 |
MAE |
1.984 |
0.803 |
1.368 |
2.025 |
1.758 |
1.026 |
MAPE |
0.266 |
0.168 |
0.329 |
0.357 |
0.565 |
0.179 |
SFE |
2.401 |
0.991 |
1.488 |
2.161 |
1.824 |
1.193 |
D+C |
Bias |
3.430 |
2.228 |
1.862 |
3.903 |
2.849 |
1.761 |
MSE |
23.483 |
9.230 |
8.949 |
27.670 |
15.222 |
5.639 |
RMSE |
4.846 |
3.038 |
2.991 |
5.260 |
3.902 |
2.375 |
MAE |
5.035 |
2.641 |
2.806 |
5.457 |
3.648 |
2.171 |
MAPE |
0.659 |
0.487 |
0.568 |
0.872 |
1.027 |
0.373 |
SFE |
3.508 |
2.117 |
2.399 |
3.613 |
2.731 |
1.633 |
All IESH All CPI-U |
D |
Bias |
-0.976 |
-0.976 |
-0.258 |
0.873 |
0.387 |
-1.487 |
MSE |
5.234 |
1.892 |
1.394 |
3.648 |
2.563 |
3.709 |
RMSE |
2.288 |
1.375 |
1.181 |
1.910 |
1.601 |
1.926 |
MAE |
1.734 |
1.261 |
0.981 |
1.663 |
1.314 |
1.842 |
MAPE |
0.203 |
0.224 |
0.216 |
0.323 |
0.449 |
0.290 |
SFE |
2.120 |
0.994 |
1.181 |
1.741 |
1.592 |
1.255 |
D+C |
Bias |
0.980 |
1.249 |
1.586 |
3.232 |
2.058 |
0.513 |
MSE |
9.101 |
5.401 |
4.235 |
19.313 |
8.263 |
2.061 |
RMSE |
3.017 |
2.324 |
2.058 |
4.395 |
2.875 |
1.436 |
MAE |
2.705 |
2.097 |
1.873 |
4.163 |
2.468 |
1.098 |
MAPE |
0.388 |
0.399 |
0.414 |
0.731 |
0.809 |
0.201 |
SFE |
2.924 |
2.008 |
1.343 |
3.051 |
2.057 |
1.374 |
All IESH 12 CPI-U |
D |
Bias |
0.408 |
-0.466 |
0.201 |
1.451 |
0.314 |
-0.889 |
MSE |
7.930 |
1.492 |
2.045 |
4.734 |
4.166 |
2.019 |
RMSE |
2.816 |
1.222 |
1.430 |
2.176 |
2.041 |
1.421 |
MAE |
2.464 |
1.009 |
1.187 |
2.049 |
1.739 |
1.251 |
MAPE |
0.332 |
0.188 |
0.273 |
0.387 |
0.537 |
0.202 |
SFE |
2.855 |
1.157 |
1.451 |
1.661 |
2.066 |
1.135 |
D+C |
Bias |
3.419 |
1.959 |
1.927 |
5.236 |
2.348 |
1.202 |
MSE |
24.619 |
8.501 |
11.953 |
36.067 |
13.339 |
3.851 |
RMSE |
4.962 |
2.916 |
3.457 |
6.006 |
3.652 |
1.962 |
MAE |
5.130 |
2.827 |
3.282 |
6.936 |
3.597 |
1.711 |
MAPE |
0.697 |
0.520 |
0.674 |
1.092 |
1.024 |
0.306 |
SFE |
3.684 |
2.212 |
2.941 |
3.015 |
2.867 |
1.590 |
All IESH 6 CPI-U |
D |
Bias |
0.422 |
-0.795 |
-0.184 |
1.300 |
0.377 |
-0.862 |
MSE |
6.056 |
2.881 |
2.509 |
4.372 |
2.899 |
2.073 |
RMSE |
2.461 |
1.697 |
1.584 |
2.091 |
1.703 |
1.440 |
MAE |
2.117 |
1.370 |
1.326 |
1.884 |
1.401 |
1.248 |
MAPE |
0.288 |
0.245 |
0.290 |
0.358 |
0.470 |
0.200 |
SFE |
2.484 |
1.537 |
1.612 |
1.678 |
1.701 |
1.182 |
D+C |
Bias |
3.250 |
0.375 |
0.873 |
4.528 |
1.984 |
0.835 |
MSE |
21.934 |
7.800 |
7.192 |
29.410 |
11.396 |
3.142 |
RMSE |
4.683 |
2.793 |
2.682 |
5.423 |
3.376 |
1.773 |
MAE |
4.768 |
2.443 |
2.427 |
5.782 |
2.963 |
1.525 |
MAPE |
0.620 |
0.456 |
0.472 |
0.926 |
0.891 |
0.269 |
SFE |
3.455 |
2.836 |
2.598 |
3.058 |
2.798 |
1.602 |
Sources: RBI, MOSPI and Authors’ calculations. |
Table A7: Directional Matches (in Per cent) in Nowcasts and Forecasts with Realised Inflation Figures |
|
Data |
GJ |
KT |
MP |
OD |
TN |
DL |
AS |
Nowcasts |
IESH |
31.6 |
57.9 |
57.9 |
63.2 |
57.9 |
68.4 |
31.6 |
IESH-BA |
31.6 |
57.9 |
57.9 |
63.2 |
57.9 |
68.4 |
31.6 |
Reg-based |
26.3 |
57.9 |
57.9 |
47.4 |
57.9 |
52.6 |
57.9 |
1 IESH 12 CPI-U |
D |
73.7 |
31.6 |
57.9 |
31.6 |
36.8 |
42.1 |
52.6 |
D+C |
68.4 |
36.8 |
63.2 |
52.6 |
31.6 |
31.6 |
52.6 |
1 IESH 6 CPI-U |
D |
63.2 |
26.3 |
57.9 |
31.6 |
57.9 |
36.8 |
47.4 |
D+C |
52.6 |
31.6 |
52.6 |
47.4 |
47.4 |
26.3 |
36.8 |
All IESH All CPI-U |
D |
57.9 |
31.6 |
36.8 |
36.8 |
42.1 |
36.8 |
47.4 |
D+C |
57.9 |
26.3 |
42.1 |
26.3 |
42.1 |
26.3 |
47.4 |
All IESH 12 CPI-U |
D |
47.4 |
31.6 |
47.4 |
42.1 |
36.8 |
42.1 |
36.8 |
D+C |
63.2 |
31.6 |
57.9 |
57.9 |
36.8 |
36.8 |
36.8 |
All IESH 6 CPI-U |
D |
31.6 |
36.8 |
52.6 |
42.1 |
52.6 |
31.6 |
57.9 |
D+C |
31.6 |
42.1 |
52.6 |
42.1 |
52.6 |
31.6 |
47.4 |
Three-months-ahead Forecasts |
IESH |
21.1 |
21.1 |
57.9 |
47.4 |
42.1 |
42.1 |
31.6 |
IESH-BA |
21.1 |
21.1 |
57.9 |
47.4 |
42.1 |
42.1 |
31.6 |
Reg-based |
57.9 |
21.1 |
57.9 |
47.4 |
42.1 |
42.1 |
63.2 |
1 IESH 12 CPI-U |
D |
42.1 |
52.6 |
47.4 |
36.8 |
52.6 |
63.2 |
42.1 |
D+C |
42.1 |
52.6 |
42.1 |
47.4 |
42.1 |
63.2 |
47.4 |
1 IESH 6 CPI-U |
D |
31.6 |
47.4 |
42.1 |
36.8 |
47.4 |
57.9 |
47.4 |
D+C |
31.6 |
57.9 |
47.4 |
47.4 |
42.1 |
47.4 |
42.1 |
All IESH All CPI-U |
D |
57.9 |
52.6 |
57.9 |
42.1 |
47.4 |
57.9 |
36.8 |
D+C |
52.6 |
47.4 |
47.4 |
42.1 |
42.1 |
63.2 |
42.1 |
All IESH 12 CPI-U |
D |
36.8 |
57.9 |
57.9 |
31.6 |
42.1 |
52.6 |
26.3 |
D+C |
47.4 |
57.9 |
52.6 |
31.6 |
42.1 |
52.6 |
31.6 |
All IESH 6 CPI-U |
D |
52.6 |
57.9 |
52.6 |
36.8 |
47.4 |
57.9 |
57.9 |
D+C |
47.4 |
57.9 |
52.6 |
47.4 |
47.4 |
57.9 |
52.6 |
One-year-ahead Forecasts |
IESH |
47.4 |
57.9 |
47.4 |
31.6 |
31.6 |
36.8 |
68.4 |
IESH-BA |
47.4 |
57.9 |
47.4 |
31.6 |
31.6 |
36.8 |
68.4 |
Reg-based |
57.9 |
47.4 |
42.1 |
63.2 |
63.2 |
26.3 |
36.8 |
1 IESH 12 CPI-U |
D |
31.6 |
47.4 |
57.9 |
57.9 |
47.4 |
68.4 |
57.9 |
D+C |
36.8 |
47.4 |
42.1 |
42.1 |
47.4 |
57.9 |
57.9 |
1 IESH 6 CPI-U |
D |
63.2 |
52.6 |
57.9 |
68.4 |
68.4 |
47.4 |
52.6 |
D+C |
52.6 |
68.4 |
63.2 |
63.2 |
63.2 |
68.4 |
42.1 |
All IESH All CPI-U |
D |
68.4 |
57.9 |
47.4 |
68.4 |
57.9 |
78.9 |
36.8 |
D+C |
63.2 |
63.2 |
47.4 |
57.9 |
52.6 |
84.2 |
36.8 |
All IESH 12 CPI-U |
D |
47.4 |
42.1 |
36.8 |
36.8 |
47.4 |
57.9 |
63.2 |
D+C |
52.6 |
42.1 |
36.8 |
26.3 |
47.4 |
52.6 |
57.9 |
All IESH 6 CPI-U |
D |
63.2 |
52.6 |
63.2 |
42.1 |
47.4 |
63.2 |
47.4 |
D+C |
57.9 |
68.4 |
68.4 |
47.4 |
42.1 |
73.7 |
31.6 |
Sources: RBI, MOSPI and Authors’ calculations. |
Table A7 (I): Directional Matches (in Per cent) in Nowcasts and Forecasts with Realised Inflation Figures (contd.) |
|
Data |
TL |
RJ |
JK |
WB |
UP |
MHM |
MHN |
Nowcasts |
IESH |
57.9 |
47.4 |
73.7 |
68.4 |
52.6 |
57.9 |
57.9 |
IESH-BA |
57.9 |
47.4 |
73.7 |
68.4 |
52.6 |
57.9 |
57.9 |
Reg-based |
52.6 |
42.1 |
0.0 |
63.2 |
47.4 |
47.4 |
52.6 |
1 IESH 12 CPI-U |
D |
47.4 |
63.2 |
47.4 |
47.4 |
57.9 |
42.1 |
42.1 |
D+C |
31.6 |
63.2 |
42.1 |
47.4 |
42.1 |
52.6 |
26.3 |
1 IESH 6 CPI-U |
D |
57.9 |
73.7 |
52.6 |
47.4 |
52.6 |
42.1 |
47.4 |
D+C |
42.1 |
68.4 |
47.4 |
52.6 |
42.1 |
36.8 |
42.1 |
All IESH All CPI-U |
D |
57.9 |
68.4 |
57.9 |
42.1 |
36.8 |
63.2 |
47.4 |
D+C |
57.9 |
52.6 |
63.2 |
36.8 |
36.8 |
52.6 |
36.8 |
All IESH 12 CPI-U |
D |
36.8 |
73.7 |
52.6 |
57.9 |
47.4 |
57.9 |
57.9 |
D+C |
26.3 |
63.2 |
47.4 |
47.4 |
42.1 |
47.4 |
52.6 |
All IESH 6 CPI-U |
D |
47.4 |
68.4 |
57.9 |
42.1 |
36.8 |
42.1 |
42.1 |
D+C |
42.1 |
63.2 |
63.2 |
36.8 |
31.6 |
31.6 |
36.8 |
Three-months-ahead Forecasts |
IESH |
47.4 |
36.8 |
26.3 |
26.3 |
42.1 |
36.8 |
57.9 |
IESH-BA |
47.4 |
36.8 |
26.3 |
26.3 |
42.1 |
36.8 |
57.9 |
Reg-based |
36.8 |
26.3 |
0.0 |
26.3 |
42.1 |
26.3 |
47.4 |
1 IESH 12 CPI-U |
D |
42.1 |
47.4 |
47.4 |
52.6 |
47.4 |
63.2 |
47.4 |
D+C |
52.6 |
47.4 |
42.1 |
57.9 |
42.1 |
57.9 |
42.1 |
1 IESH 6 CPI-U |
D |
57.9 |
36.8 |
42.1 |
52.6 |
57.9 |
57.9 |
36.8 |
D+C |
63.2 |
36.8 |
52.6 |
52.6 |
63.2 |
68.4 |
57.9 |
All IESH All CPI-U |
D |
42.1 |
26.3 |
47.4 |
52.6 |
52.6 |
42.1 |
63.2 |
D+C |
42.1 |
36.8 |
47.4 |
57.9 |
57.9 |
57.9 |
68.4 |
All IESH 12 CPI-U |
D |
52.6 |
36.8 |
57.9 |
31.6 |
47.4 |
42.1 |
42.1 |
D+C |
52.6 |
42.1 |
42.1 |
42.1 |
42.1 |
47.4 |
47.4 |
All IESH 6 CPI-U |
D |
57.9 |
31.6 |
42.1 |
42.1 |
52.6 |
52.6 |
57.9 |
D+C |
63.2 |
31.6 |
47.4 |
47.4 |
63.2 |
68.4 |
68.4 |
One-year-ahead Forecasts |
IESH |
52.6 |
26.3 |
15.8 |
42.1 |
36.8 |
36.8 |
31.6 |
IESH-BA |
52.6 |
26.3 |
15.8 |
42.1 |
36.8 |
36.8 |
31.6 |
Reg-based |
57.9 |
36.8 |
15.8 |
52.6 |
57.9 |
52.6 |
52.6 |
1 IESH 12 CPI-U |
D |
63.2 |
63.2 |
89.5 |
52.6 |
57.9 |
63.2 |
57.9 |
D+C |
73.7 |
63.2 |
84.2 |
52.6 |
57.9 |
57.9 |
68.4 |
1 IESH 6 CPI-U |
D |
42.1 |
73.7 |
78.9 |
36.8 |
57.9 |
42.1 |
52.6 |
D+C |
47.4 |
57.9 |
68.4 |
42.1 |
57.9 |
52.6 |
57.9 |
All IESH All CPI-U |
D |
36.8 |
21.1 |
73.7 |
47.4 |
52.6 |
57.9 |
63.2 |
D+C |
42.1 |
36.8 |
73.7 |
57.9 |
42.1 |
68.4 |
73.7 |
All IESH 12 CPI-U |
D |
73.7 |
36.8 |
78.9 |
52.6 |
52.6 |
57.9 |
57.9 |
D+C |
73.7 |
52.6 |
68.4 |
47.4 |
57.9 |
57.9 |
57.9 |
All IESH 6 CPI-U |
D |
36.8 |
42.1 |
57.9 |
36.8 |
57.9 |
47.4 |
47.4 |
D+C |
42.1 |
47.4 |
63.2 |
47.4 |
57.9 |
47.4 |
47.4 |
Sources: RBI, MOSPI and Authors’ calculations. |
Table A7 (II): Directional Matches (in Per cent) in Nowcasts and Forecasts with Realised Inflation Figures (contd.) |
|
Data |
BR |
KL |
CH |
JH |
CHH |
AC |
Nowcasts |
IESH |
52.6 |
73.7 |
52.6 |
21.1 |
42.1 |
57.9 |
IESH-BA |
52.6 |
73.7 |
52.6 |
21.1 |
42.1 |
57.9 |
Reg-based |
47.4 |
68.4 |
36.8 |
21.1 |
26.3 |
68.4 |
1 IESH 12 CPI-U |
D |
47.4 |
63.2 |
47.4 |
63.2 |
21.1 |
42.1 |
D+C |
68.4 |
47.4 |
57.9 |
42.1 |
36.8 |
42.1 |
1 IESH 6 CPI-U |
D |
47.4 |
63.2 |
47.4 |
52.6 |
31.6 |
52.6 |
D+C |
47.4 |
52.6 |
63.2 |
52.6 |
31.6 |
36.8 |
All IESH All CPI-U |
D |
36.8 |
63.2 |
68.4 |
52.6 |
36.8 |
42.1 |
D+C |
42.1 |
42.1 |
52.6 |
47.4 |
42.1 |
47.4 |
All IESH 12 CPI-U |
D |
31.6 |
52.6 |
47.4 |
42.1 |
47.4 |
47.4 |
D+C |
31.6 |
52.6 |
57.9 |
31.6 |
57.9 |
57.9 |
All IESH 6 CPI-U |
D |
36.8 |
68.4 |
68.4 |
63.2 |
26.3 |
47.4 |
D+C |
47.4 |
57.9 |
63.2 |
42.1 |
36.8 |
36.8 |
Three-months-ahead Forecasts |
IESH |
68.4 |
52.6 |
57.9 |
47.4 |
52.6 |
26.3 |
IESH-BA |
68.4 |
52.6 |
57.9 |
47.4 |
52.6 |
26.3 |
Reg-based |
57.9 |
47.4 |
31.6 |
42.1 |
47.4 |
15.8 |
1 IESH 12 CPI-U |
D |
15.8 |
57.9 |
57.9 |
68.4 |
57.9 |
52.6 |
D+C |
31.6 |
47.4 |
63.2 |
42.1 |
68.4 |
57.9 |
1 IESH 6 CPI-U |
D |
31.6 |
57.9 |
52.6 |
52.6 |
63.2 |
47.4 |
D+C |
42.1 |
52.6 |
52.6 |
42.1 |
73.7 |
52.6 |
All IESH All CPI-U |
D |
47.4 |
42.1 |
63.2 |
36.8 |
57.9 |
52.6 |
D+C |
36.8 |
42.1 |
52.6 |
47.4 |
52.6 |
47.4 |
All IESH 12 CPI-U |
D |
31.6 |
47.4 |
63.2 |
42.1 |
52.6 |
36.8 |
D+C |
42.1 |
36.8 |
57.9 |
47.4 |
52.6 |
47.4 |
All IESH 6 CPI-U |
D |
42.1 |
31.6 |
57.9 |
47.4 |
63.2 |
42.1 |
D+C |
36.8 |
31.6 |
52.6 |
42.1 |
63.2 |
47.4 |
One-year-ahead Forecasts |
IESH |
57.9 |
26.3 |
31.6 |
36.8 |
63.2 |
26.3 |
IESH-BA |
57.9 |
26.3 |
31.6 |
36.8 |
63.2 |
26.3 |
Reg-based |
42.1 |
73.7 |
63.2 |
52.6 |
57.9 |
52.6 |
1 IESH 12 CPI-U |
D |
42.1 |
52.6 |
52.6 |
52.6 |
52.6 |
47.4 |
D+C |
42.1 |
42.1 |
63.2 |
47.4 |
47.4 |
47.4 |
1 IESH 6 CPI-U |
D |
57.9 |
63.2 |
47.4 |
57.9 |
68.4 |
52.6 |
D+C |
63.2 |
42.1 |
68.4 |
47.4 |
78.9 |
52.6 |
All IESH All CPI-U |
D |
68.4 |
42.1 |
52.6 |
52.6 |
68.4 |
57.9 |
D+C |
42.1 |
36.8 |
52.6 |
57.9 |
52.6 |
63.2 |
All IESH 12 CPI-U |
D |
36.8 |
42.1 |
52.6 |
57.9 |
57.9 |
47.4 |
D+C |
31.6 |
47.4 |
52.6 |
52.6 |
57.9 |
31.6 |
All IESH 6 CPI-U |
D |
63.2 |
26.3 |
52.6 |
47.4 |
68.4 |
31.6 |
D+C |
47.4 |
26.3 |
52.6 |
47.4 |
63.2 |
42.1 |
Sources: RBI, MOSPI and Authors’ calculations. |
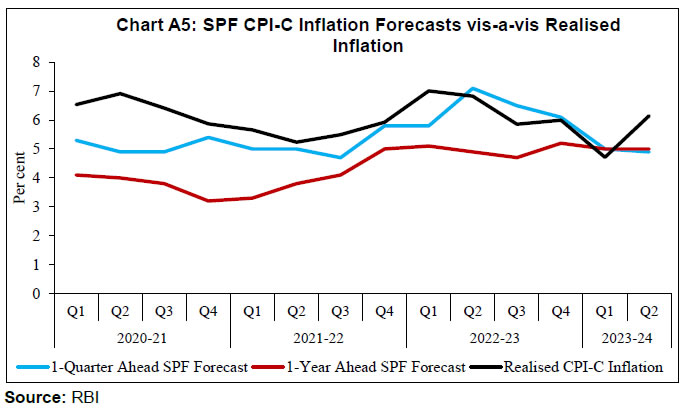
Table A8: Performance of SPF Forecasts vis-à-vis Forecasts from Proposed Methodology |
Measures of Forecast Error |
1-Quarter Ahead SPF Forecast |
Three-months-ahead Proposed Forecast |
1-Year Ahead SPF Forecast |
One-year-ahead Proposed Forecast |
Bias |
-0.587 |
0.024 |
-1.672 |
0.266 |
MSE |
0.919 |
1.002 |
3.544 |
1.228 |
RMSE |
0.959 |
1.001 |
1.883 |
1.108 |
MAE |
0.773 |
0.861 |
1.713 |
0.947 |
MAPE |
0.124 |
0.154 |
0.278 |
0.166 |
Theil's U |
0.029 |
0.084 |
0.070 |
0.097 |
SFE |
0.575 |
1.025 |
0.747 |
1.084 |
Directional Matches (in per cent) |
38.5 |
52.6 |
76.9 |
63.2 |
Sources: RBI, MOSPI and Authors’ calculations. |
|